Synth pads: how to create atmospheric textures in your music
What are synth pads?
A synth pad is a sustained, pitched sound typically created using a synthesizer. It could be a single note or a chord (two or more notes at once). Synth pads tend to have a long loudness envelope, meaning they fade in gently, stick around for a while, and trail away slowly when their moment has passed. Synth pads are smooth, atmospheric sounds that play an important supporting role in many musical contexts.
We can understand pads better by comparing them with other synthesizer elements you might hear in electronic music. A synth lead is more of a star player: it sits in the foreground of a track, outlining a single melody or topline. This might be the part you hum along to when listening to the track. A synth arpeggio, meanwhile, is a rhythmic sequence of notes that can be used to create movement and energy.
Synth pads, by contrast, don’t supply melody or add rhythmic movement to a track. Instead, they add atmosphere and depth, helping to fill out an arrangement and give your music richness and shine. Synth pads are used in all kinds of music, from pop and electronic tracks through to film and video game scores.
Sub bass: how to add that deep tone to your tracks
Final pro tips for sub bass
Whether you have your sub bass follow along with your bass line, use it only to accentuate certain notes, or let it steal the show by occupying the entire low end, here are some of my top pro tips for using sub bass in your productions.
Layer your sub bass
Sometimes, one sub bass isn’t enough to achieve the desired impact. Layering different sub bass sounds can add depth and complexity. For example, layering a clean sine wave with a subtly distorted triangle wave can combine the best of both worlds: solid low-end power with a hint of harmonic interest. When layering, pay close attention to phase alignment and check your EQs to make sure the layers complement rather than conflict with each other.
Use sidechain compression
Sidechain compression is a powerful technique to ensure your sub bass and kick drum coexist harmoniously in the mix, each with its own clear space. By applying sidechain compression to your sub bass, triggered by the kick drum, the sub bass volume temporarily ducks each time the kick hits. This creates a rhythmic pulse that not only prevents frequency clashes but also adds a dynamic movement to your track, enhancing the groove.
Monitor your volume levels
Volume leveling is a crucial step in integrating your sub bass seamlessly into the mix, ensuring it provides a solid foundation without overshadowing the other musical elements. By carefully adjusting the volume of your sub bass, you can maintain a balance that complements the overall track, allowing it to support the mix rather than dominate it.
Use automation
For parts of your track that might have varying energy levels or where the sub bass needs to be more prominent or subdued, volume automation becomes an invaluable tool. This technique allows for precise control over the sub bass volume throughout different sections of your song, adapting in real-time to the dynamic needs of the track to keep the energy consistent and engaging.
Play with spatial placement
While sub bass frequencies inherently possess an omnidirectional quality, making them feel like they’re coming from everywhere, a strategic approach to spatial placement can elevate your track. By applying slight stereo widening to the upper harmonics of your sub bass, you introduce an element of spatial intrigue without compromising the core low-end focus. However, it’s important to tread lightly with stereo effects on low frequencies – overdoing it can lead to phase issues that muddy your mix or weaken the impact of your sub bass when played on mono systems, such as club PA setups.
Reference and test
Ensuring your sub bass performs consistently across a variety of listening environments is crucial for its effectiveness in your mix. Reference and test your track on different systems – these can range from high-quality studio monitors to basic headphones and even built-in smartphone speakers. This will show you how your sub bass translates in real-world scenarios, highlighting any issues with balance, clarity, or presence that might not be apparent in the studio. By making adjustments based on these tests, you can achieve a sub bass that maintains its impact, whether it’s felt on a club sound system or heard through earbuds during a morning commute.
Meet the new Push | Ableton
The new Push is a standalone instrument that invites you to step away from your computer and be fully in the moment with your music. Play expressive MPE-enabled pads in your own style, and plug your other hardware straight into Push’s built-in audio interface. And as an upgradeable instrument with replaceable components, it’s set for a long life at the center of your setup.
AI and Music-Making Part 1: The State of Play
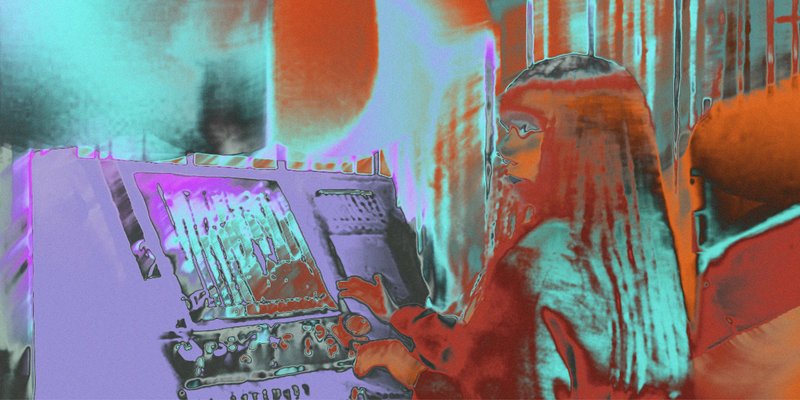
Listen to this article.
This is Part 1 of two-part aricle. Read Part 2 here.
The word “AI” provokes mixed emotions. It can inspire excitement and hope for the future - or a shiver of dread at what’s to come. In the last few years, AI has gone from a distant promise to a daily reality. Many of us use ChatGPT to write emails and Midjourney to generate images. Each week, it seems, a new AI technology promises to change another aspect of our lives.
Music is no different. AI technology is already being applied to audio, performing tasks from stem separation to vocal deepfakes, and offering new spins on classic production tools and music-making interfaces. One day soon, AI might even make music all by itself.
The arrival of AI technologies has sparked heated debates in music communities. Ideas around creativity, ownership, and authenticity are being reexamined. Some welcome what they see as exciting new tools, while others say the technology is overrated and won’t change all that much. Still others are scared, fearing the loss of the music-making practices and cultures they love.
In this two-part article, we will take a deep dive into AI music-making to try to unpick this complex and fast-moving topic. We’ll survey existing AI music-making tools, exploring the creative possibilities they open up and the philosophical questions they pose. And we will try to look ahead, examining how AI tools might change music-making in the future.
The deeper you go into the topic, the stronger those mixed emotions become. The future might be bright, but it’s a little scary too.
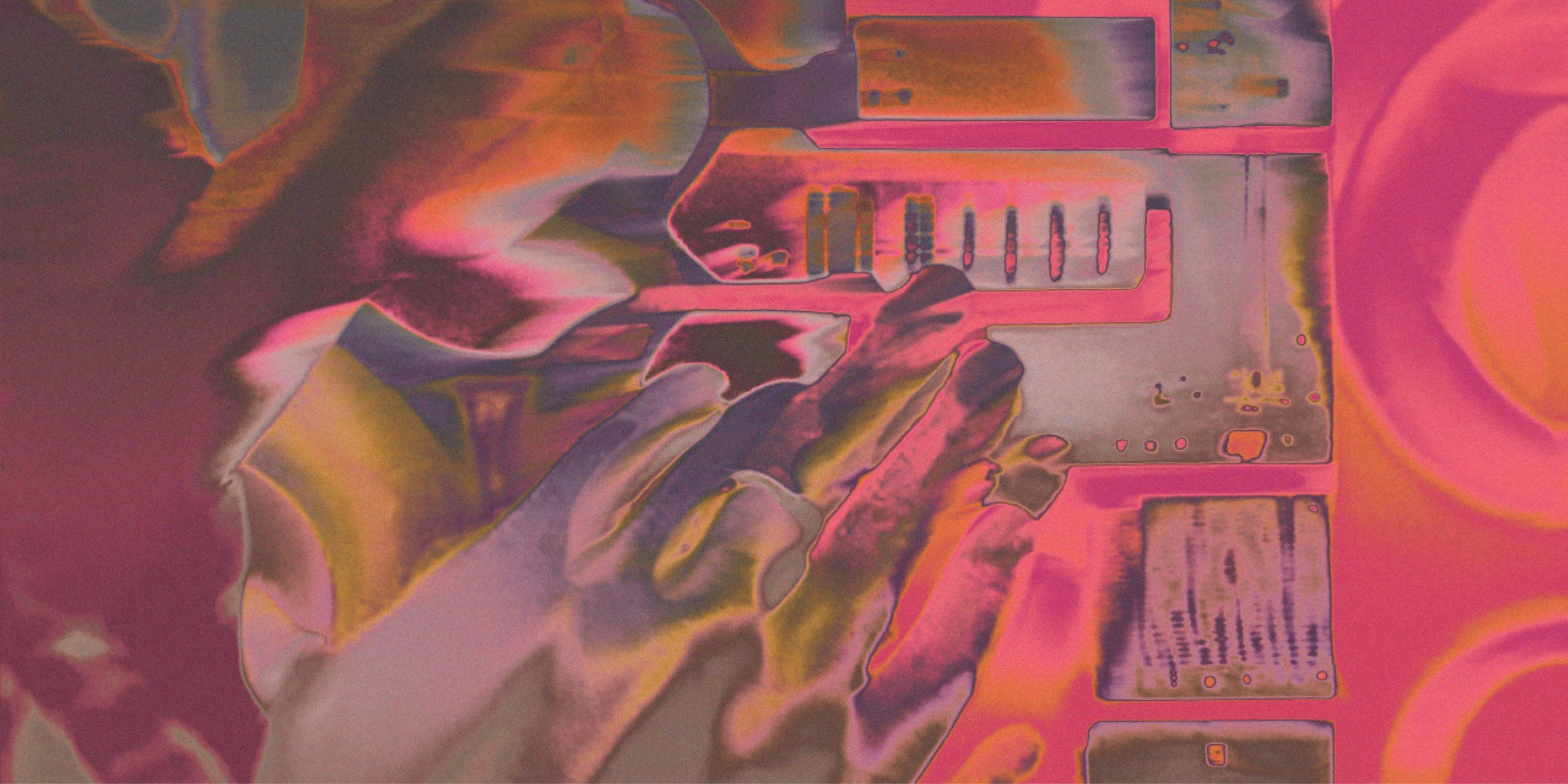
Defining terms
Before we go any further, we should get some terms straight.
First of all, what is AI? The answer isn’t as simple as you might think. Coined in the 1950s, the term has since been applied to a range of different technologies. In its broadest sense, AI refers to many forms of computer program that seem to possess human-like intelligence, or that can do tasks that we thought required human intelligence.
The AI boom of the last few years rests on a specific technology called machine learning. Rather than needing to be taught entirely by human hand, a machine learning system is able to improve itself using the data it’s fed. But machine learning has been around for decades. What’s new now is a specific kind of machine learning called deep learning.
Deep learning systems are made up of neural networks: a set of algorithms roughly configured like a human brain, that can interpret incoming data and recognize patterns. The “deep” part tells us that there are multiple layers to these networks, allowing the system to interpret data in more sophisticated ways. This makes a deep learning system very skilled at making sense of unstructured data. In other words, you can throw random pictures or text at it and it will do a good job of spotting the patterns.
But deep learning systems aren’t “intelligent” in the way often depicted in dystopian sci-fi movies about runaway AIs. They don’t possess a “consciousness” as we would understand it - they are just very good at spotting the patterns in data. For this reason, some argue that the term “AI” is a misnomer.
The sophistication of deep learning makes it processor-hungry, hence the technology only becoming widely accessible in the last few years. But deep learning technology has been present in our lives for longer, and in more ways, than you might think. Deep learning is used in online language translators, credit card fraud detection, and even the recommendation algorithms in music streaming services.
These established uses of deep learning AI mostly sit under the hood of products and services. Recently, AI has stepped into the limelight. Tools such as Dall-E and ChatGPT don’t just sift incoming data to help humans recognise the patterns. They produce an output that attempts to guess what the data will do next. This is called generative AI.
Where other forms of deep learning chug along in the background of daily life, generative AI draws attention to itself. By presenting us with images, text, or other forms of media, it invites us into a dialogue with the machine. It mirrors human creativity back at us, and makes the potentials - and challenges - of AI technology more starkly clear.
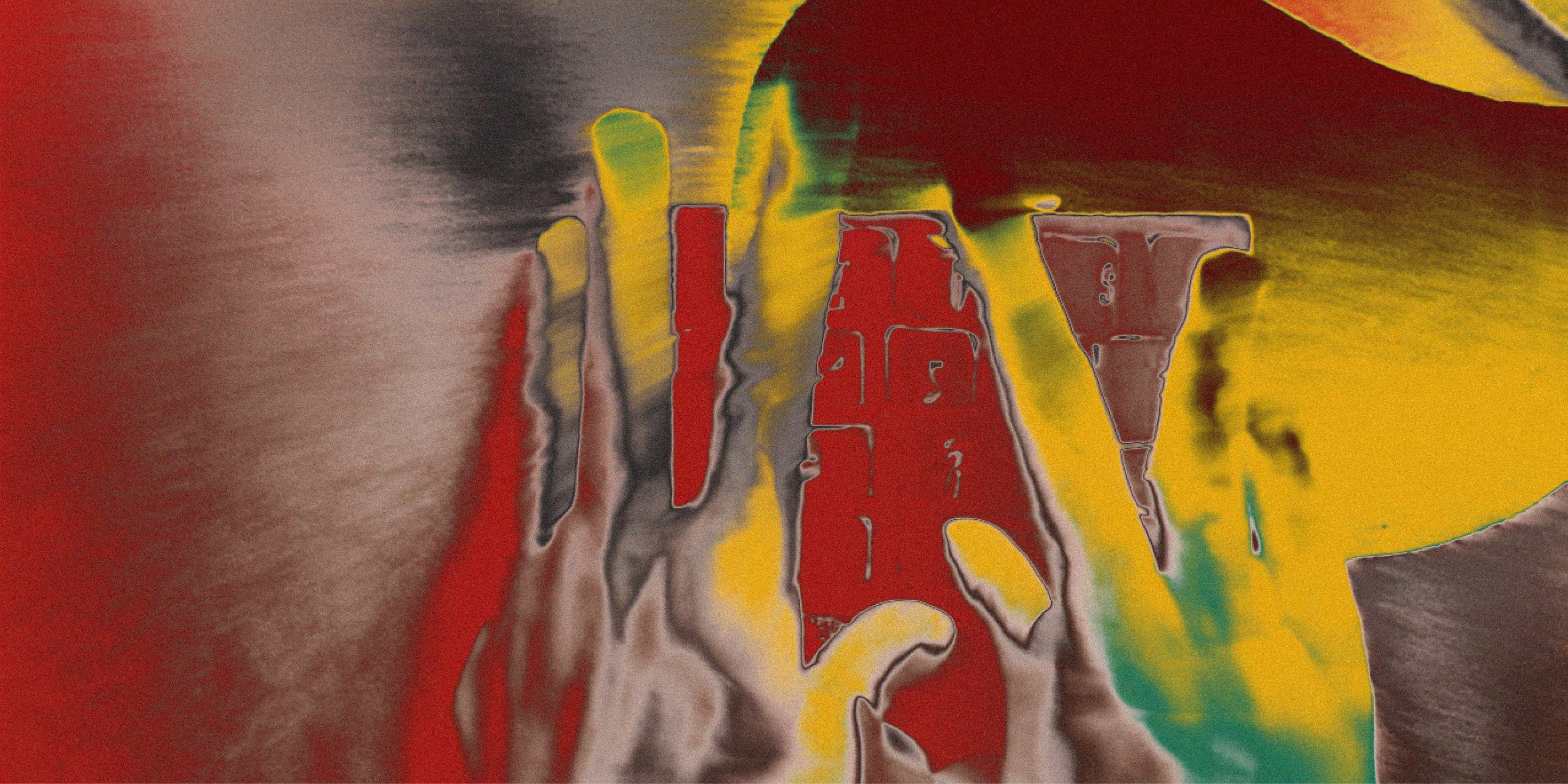
No ChatGPT for music?
Deep learning technology can be applied to digital audio just as it can to images, text, and other forms of data. The implications of this are wide-ranging, and we’ll explore them in depth in these articles. But AI audio is lagging behind some other applications of the technology. There is, as yet, no ChatGPT for music. That is: there’s no tool trained on massive amounts of audio that can accept text or other kinds of prompts and spit out appropriate, high-quality music. (Although there may be one soon - more on this in Part 2).
There are a few possible reasons for this. First of all, audio is a fundamentally different kind of data to image or text, as Christian Steinmetz, an AI audio researcher at Queen Mary University, explains. “[Audio] has relatively high sample rate - at each point in time you get one sample, assuming it’s monophonic audio. But you get 44,000 of those samples per second.” This means that generating a few minutes of audio is the data equivalent of generating an absolutely enormous image.
As AI audio researchers and innovators the Dadabots observe, this puts a limit on how fast currently available systems can work. “Some of the best quality methods of generating raw audio can require up to a day to generate a single song.”
Unlike images or text, audio has a time dimension. It matters to us how the last minute of a song relates to the first minute, and this poses specific challenges to AI. Music also seems harder to reliably describe in words, making it resistant to the text-prompt approach that works so well for images. “Music is one of our most abstract artforms,” say the Dadabots. “The meaning of timbres, harmonies, rhythms alone are up to the listener's interpretation. It can be very hard to objectively describe a full song in a concise way where others can instantly imagine it.”
Added to this, our auditory perception seems to be unusually finely tuned. “We may be sensitive to distortions in sound in a different way than our visual system is sensitive,” says Steinmetz. He gives the example of OpenAI’s Jukebox, a generative music model launched in 2020 - the most powerful at the time. It could create “super convincing music” in the sense that the important elements were there. “But it sounded really bad from a quality perspective. It’s almost as if for audio, if everything is not in the exact right place, even an untrained listener is aware that there's something up. But for an image it seems like you can get a lot of the details mostly right, and it's fairly convincing as an image. You don't need to have every pixel exactly right.”
It’s tempting to conclude that music is simply too hard a nut to crack: too mysterious, too ephemeral an aesthetic experience to be captured by the machines. That would be naive. In fact, efforts to design effective AI music tools have been progressing quickly in recent years.
There is a race on to create a “general music model” - that is, a generative music AI with a versatility and proficiency equivalent to Stable Diffusion or ChatGPT. We will explore this, and its implications for music-making, in Part 2 of this series.
But there are many potential uses for AI in music beyond this dream of a single totalizing system. From generative MIDI to whacky-sounding synthesis, automated mixing to analog modeling, AI tools have the potential to shake up the music-making process. In Part 1, we’ll explore some of what’s out there now, and get a sense of how these tools might develop in the future. In the process, we’ll address what these tools might mean for music-making. Does AI threaten human creativity, or simply augment it? Which aspects of musical creation might change, and which will likely stay the same?
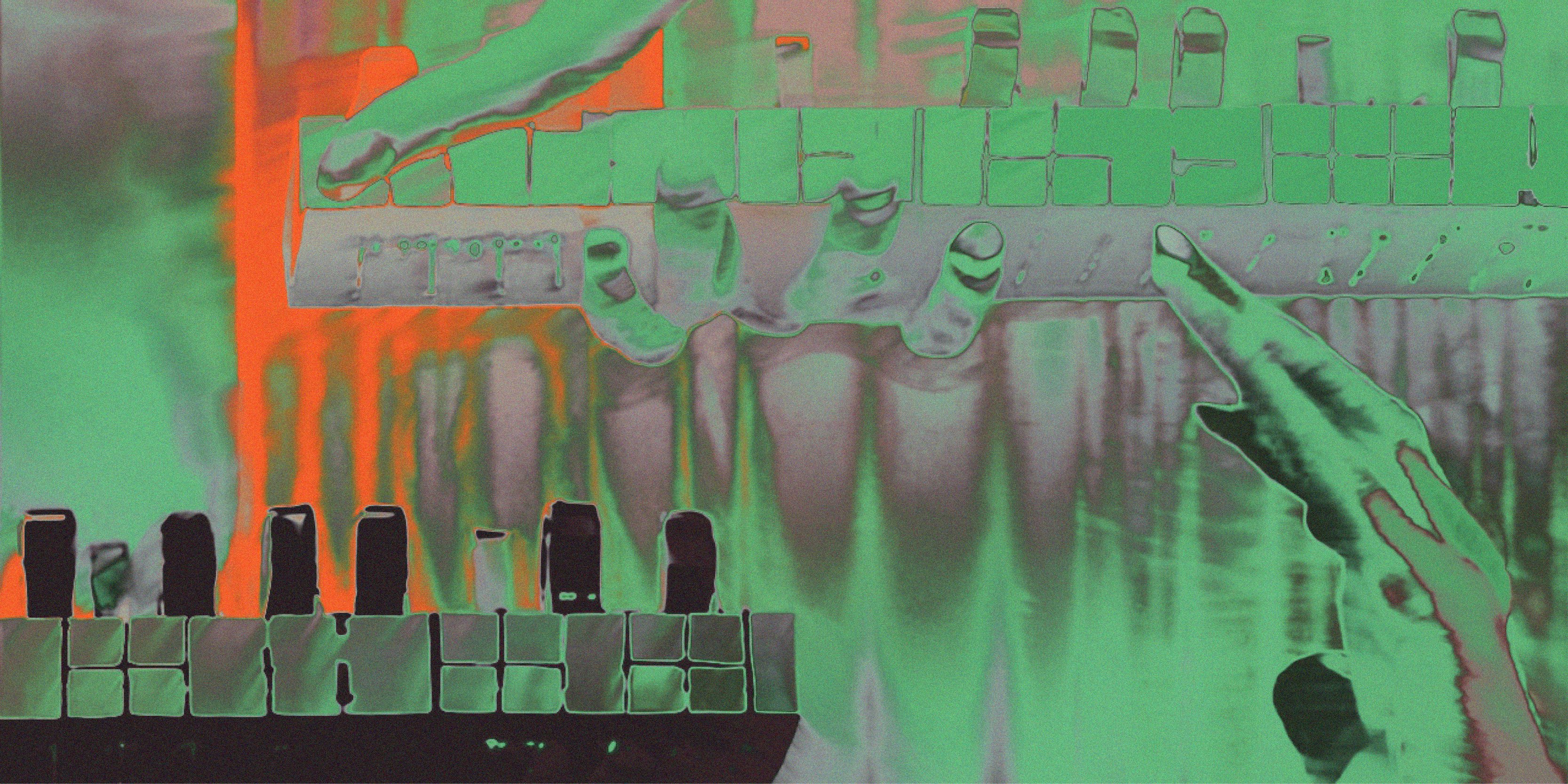
Automating production tasks
At this point you may be confused. If you are a music producer or other audio professional, “AI music production tools” might not seem like such a novel idea. In fact, the “AI” tag has been floating around in the music tech world for years.
For example, iZotope have integrated AI into products like their all-in-one mixing tool, Neutron 4. The plug-in’s Mix Assistant listens to your whole mix and analyzes the relationships between the sounds, presenting you with an automated mix that you can tweak to taste.
Companies like Sonible, meanwhile, offer “smart” versions of classic plug-in effects such as compression, reverb, and EQ. These plug-ins listen to the incoming audio and adapt to it automatically. The user is then given a simpler set of macro controls for tweaking the settings. pure:comp, for instance, offers just one main “compression” knob that controls parameters such as threshold, ratio, attack, and release simultaneously.
Other tools offer to automate parts of the production process that many producers tend to outsource. LANDR will produce an AI-automated master of your track for a fraction of the cost of hiring a professional mastering engineer. You simply upload your premaster to their website, choose between a handful of mastering styles and loudness levels, and download the mastered product.
What is the relationship between these tools and the deep learning technologies that are breaking through now? Here we come back to the vagueness of the term “AI.” Deep learning is one kind of AI technology, but it’s not the only one. Before that, we had “expert systems.”
As Steinmetz explains, this method works “by creating a tree of options.” He describes how an automated mixing tool might work following this method. “If the genre is jazz, then you go to this part of the tree. If it’s jazz and the instrument is an upright bass, then you go to this part of the tree. If it's an upright bass and there's a lot of energy at 60 hertz, then maybe decrease that. You come up with a rule for every possible scenario. If you can build a complicated enough set of rules, you will end up with a system that appears intelligent.”
"If you're doing a job that could theoretically be automated - meaning that no one cares about the specifics of the artistic outputs, we just need it to fit some mold - then that job is probably going to be automated eventually."
It’s difficult to say for sure what technology is used in individual products. But it’s likely that AI-based music tech tools that have been around for more than a few years use some variation of this approach. (Of course, deep learning methods may have been integrated into these tools more recently).
This approach is effective when executed well, but it has limitations. As Steinmetz explains, such technology requires expert audio engineers to sit down with programmers and write all the rules. And as anyone who has mixed a track will know, it’s never so simple as following the rules. A skilled mix engineer makes countless subtle decisions and imaginative moves. The number of rules you’d need to fully capture this complexity is simply too vast. “The problem is of scale, basically,” says Steinmetz.
Here’s where deep learning comes in. Remember: deep learning systems can teach themselves from data. They don’t need to be micromanaged by a knowledgeable human. The more relevant data they’re fed, and the more processor power they have at their disposal, the more proficient they can become at their allotted task.
This means that a deep learning model fed on large amounts of music would likely do a better job than an expert systems approach - and might, by some metrics, even surpass a human mix engineer.
This is not yet a reality in the audio domain, but Steinmetz points to image classification as an example of AI tools reaching this level. “The best model is basically more accurate than a human at classifying the contents of an image, because we've trained it on millions of images - more images than a human would even be able to look at. So that's really powerful.”
This means that AI will probably get very good at various technical tasks that music producers have until now considered an essential part of the job. From micro-chores like setting your compressor’s attack and decay, to diffuse tasks like finalizing your entire mixdown, AI may soon be your very own in-house engineer.
How will this change things for music-makers? Steinmetz draws an analogy with the democratization of digital photography through smartphone cameras. Professional photographers who did workaday jobs like documenting events lost out; demand for fine art photographers stayed the same.
“In mixing or audio engineering, it's a similar thing. If you're doing a job that could theoretically be automated - meaning that no one cares about the specifics of the artistic outputs, we just need it to fit some mold - then that job is probably going to be automated eventually.” But when a creative vision is being realized, the technology won’t be able to replace the decision-maker. Artists will use “the AI as a tool, but they're still sitting in the pilot's seat. They might let the tool make some decisions, but at the end of the day, they're the executive decision-maker.”
Of course, this won’t be reassuring to those who make their living exercising their hard-won production or engineering skills in more functional ways. We might also wonder whether the next generation of producers could suffer for it. There is a creative aspect to exactly how you compress, EQ, and so on. If technology automates these processes, will producers miss out on opportunities to find creative new solutions to age-old problems - and to make potentially productive mistakes?
On the other hand, by automating these tasks, music-makers will free up time and energy - which they can spend expanding the creative scope of their music in other ways. Many tasks that a current DAW executes in seconds would, in the era of analog studios, have taken huge resources, work hours, and skill. We don’t consider the music made on modern DAWs to be creatively impoverished as a result. Instead, the locus of creativity has shifted, as new sounds, techniques, and approaches have become accessible to more and more music-makers.
“It is true that some aspects of rote musical production are likely to be displaced by tools that might make light work of those tasks,” says Mat Dryhurst, co-founder - alongside his partner, the musician Holly Herndon - of the AI start-up Spawning. “But that just shifts the baseline for what we consider art to be. Generally speaking artists we cherish are those that deviate from the baseline for one reason or another, and there will be great artists in the AI era just as there have been great artists in any era.”
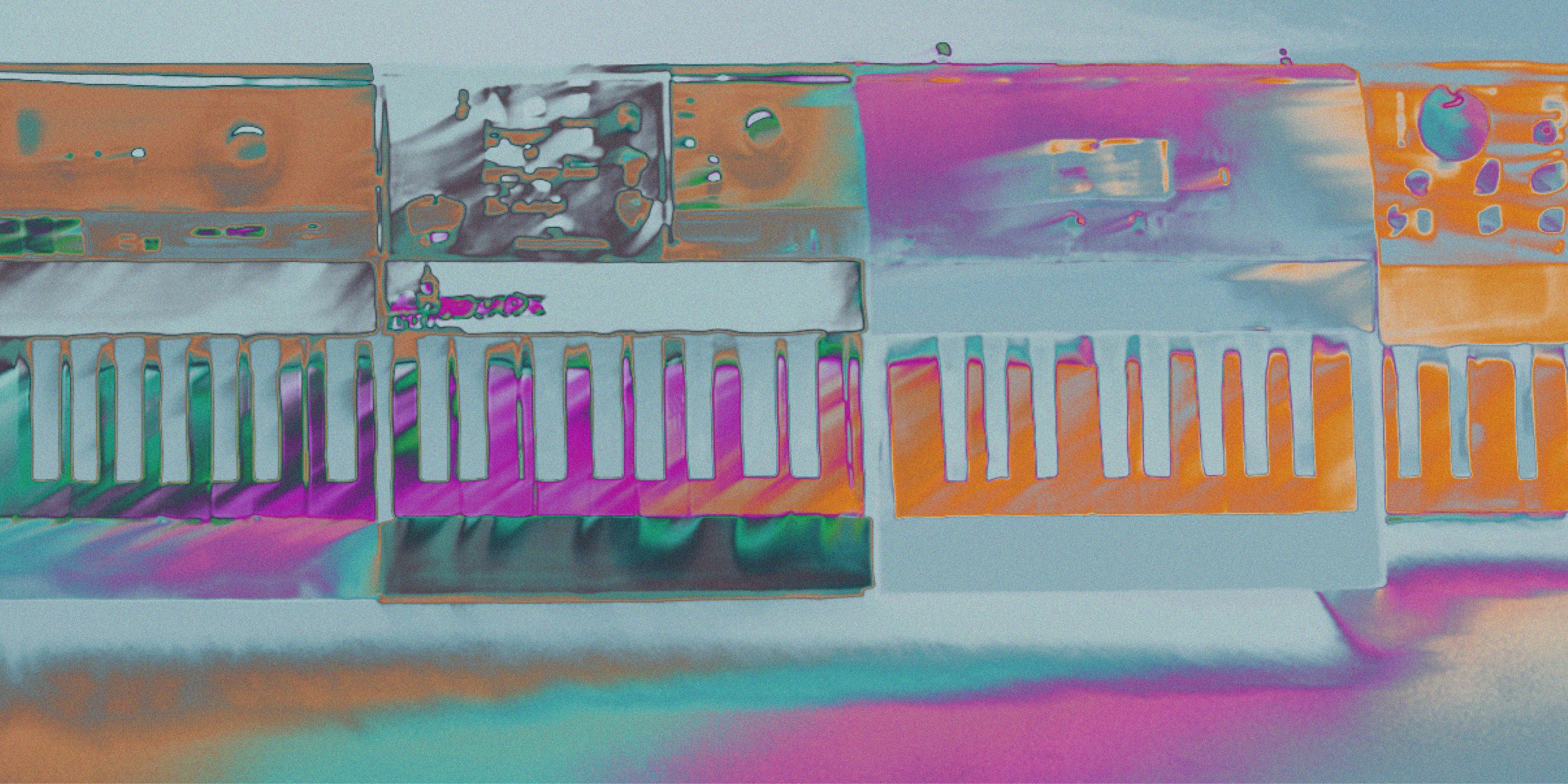
In the beginning there was MIDI
Making a distinction between functional production tasks and artistry is relatively easy when thinking about technical tasks such as mixing. But what about the composition side? AI could shake things up here too.
An early attempt to apply machine learning in this field was Magenta Studio, a project from Google’s Magenta research lab that was made available as a suite of Max For Live tools in 2019. These tools offer a range of takes on MIDI note generation: creating a new melody or rhythm from scratch; completing a melody based on notes given; “morphing” between two melodic clips. Trained on “millions” of melodies and rhythms, these models offer a more sophisticated - and, perhaps, more musical - output than traditional generative tools.
AI-powered MIDI note generation has been taken further by companies like Orb Plugins, who have packaged the feature into a set of conventional soft synths – similar to Mixed In Key's Captain plug-ins. Drum sequencers, meanwhile, have begun to incorporate the technology to offer users rhythmic inspiration.
Why the early interest in MIDI? MIDI notation is very streamlined data compared to audio’s 44,000 samples per second, meaning models can be simpler and run lighter. When the technology was in its infancy, MIDI was an obvious place to start.
Of course, MIDI’s compactness comes with limitations. Pitches and rhythms are only part of music’s picture. Addressing the preference for MIDI among machine learning/music hackers a few years ago, the Dadabots wrote: “MIDI is only 2% of what there is to love about music. You can’t have Merzbow as MIDI. Nor the atmosphere of a black metal record. You can’t have the timbre of Jimi Hendrix’s guitar, nor Coltrane’s sax, nor MC Ride. Pure MIDI is ersatz.”
As AI technology gets more sophisticated and processor power increases, tools are emerging that allow musicians to work directly with raw audio. So are MIDI-based AI tools already a thing of the past?
Probably not. Most modern musicians rely on MIDI and other “symbolic” music languages. Electronic producers punch rhythms into a sequencer, draw notes in the piano roll, and draw on techniques grounded in music theory traditions (such as keys and modes). AI can offer a lot here. Besides generating ideas, we could use MIDI-based AI tools to accurately transcribe audio into notation, and to perform complex transformations of MIDI data. (For instance, transforming rhythms or melodies from one style or genre into another).
In a talk arguing for the continued importance of “symbolic music generation,” Julian Lenz of AI music company Qosmo pointed out that raw audio models aren’t yet good at grasping the basics of music theory. For example, Google’s MusicLM, a recent general music model trained on hundreds of thousands of audio clips, has trouble distinguishing between major and minor keys. Lenz concluded by demonstrating a new Qosmo plugin that takes a simple tapped rhythm and turns it into a sophisticated, full-kit drum performance. While raw audio AI tools remain somewhat janky, MIDI-based tools may offer quicker routes to inspiration.
Such tools pose tricky questions about the attribution of creativity. If an AI-based plug-in generates a melody for you, should you be considered the “composer” of that melody? What if you generated the melody using an AI model trained on songs by the Beatles? Is the melody yours, the AI’s, or should the Beatles get the credit?
These questions apply to many forms of AI music-making, and we’ll return to them in Part 2. For now it’s sufficient to say that, when it comes to MIDI-based melody and rhythm generation, the waters of attribution have been muddied for a long time. Modern electronic composers often use note randomizers, sophisticated arpeggiators, Euclidean rhythm generators, and so on. The generated material is considered a starting point, to be sifted, edited, and arranged according to the music-maker’s creative vision. AI tools may give us more compelling results straight out the gate. But a human subjectivity will still need to decide how the generated results fit into their creative vision.
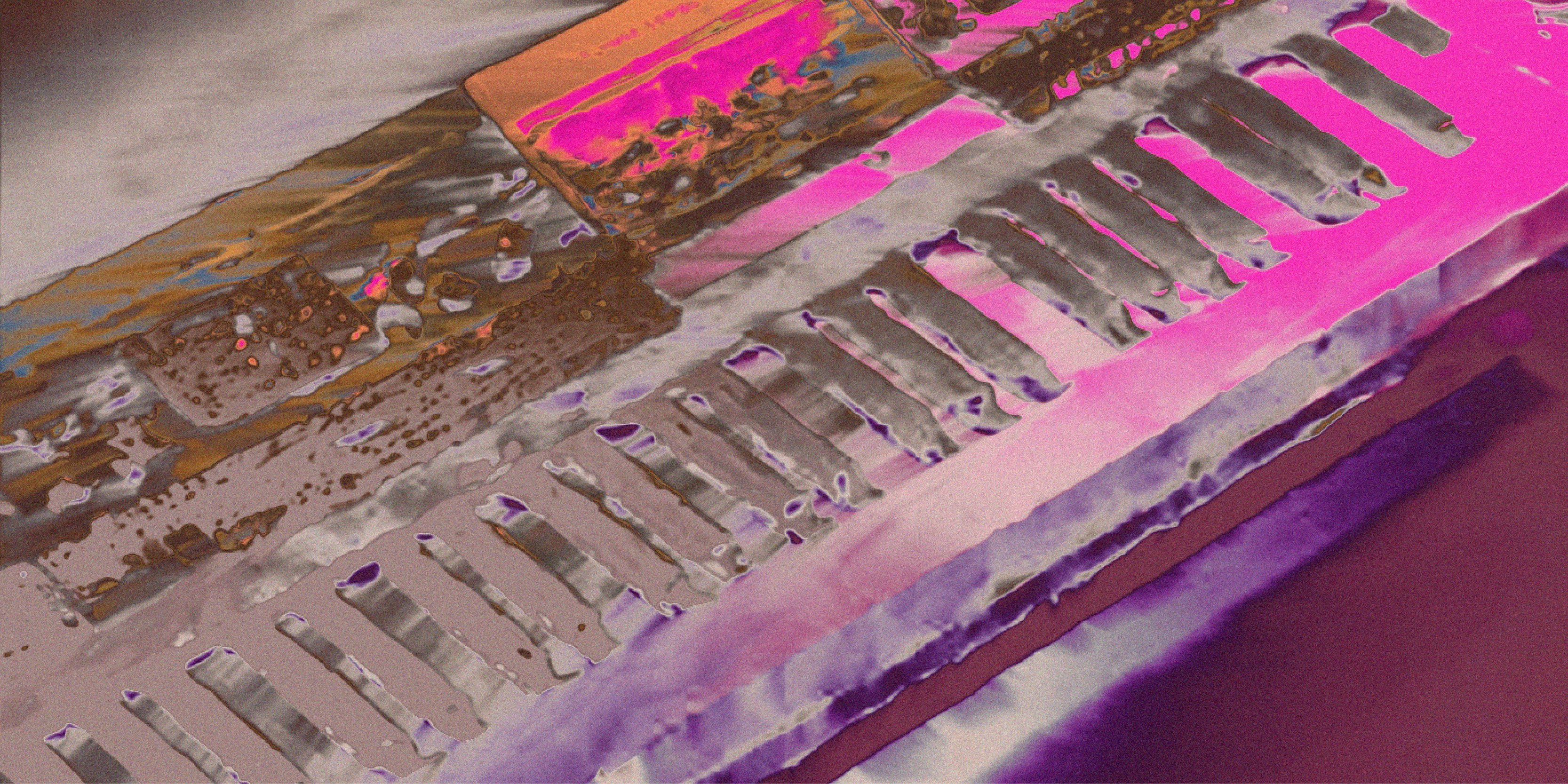
Timbre transfer: Exploring new sounds
When we think of a radical new technology like AI, we might imagine wild new sounds and textures. MIDI is never going to get us there. For this, we need to turn to the audio realm.
In the emerging field of “neural synthesis,” one of the dominant technologies is timbral transfer. Put simply, timbral transfer takes an audio input and makes it sound like something else. A voice becomes a violin; a creaking door becomes an Amen break.
How does this work? Timbre transfer models, such as IRCAM’s RAVE (“Realtime Audio Variational autoEncoder”), feature two neural networks working in tandem. One encodes the audio it receives, capturing it according to certain parameters (like loudness or pitch). Using this recorded data, the other neural net then tries to reconstruct (or decode) the input.
The sounds that an autoencoder spits out depend on the audio it’s been trained on. If you’ve trained it on recordings of a flute, then the decoder will output flute-like sounds. This is where the “timbre transfer” part comes in. If you feed your flute-trained encoder a human voice, it will still output flute sounds. The result is a strange amalgam: the contours of the voice with the timbre of a flute.
Timbre transfer is already available in a number of plug-ins, though none have yet been presented to the mass market. Perhaps the most accessible is Qosmo’s Neutone, a free-to-download plug-in that allows you to try out a number of neural synthesis techniques in your DAW. This includes RAVE and another timbre transfer method called DDSP (Differentiable Digital Signal Processing). DDSP is a kind of hybrid of the encoder technology and the DSP found in conventional synthesis. It’s easier to train and can give better-sounding outputs - providing the input audio is monophonic.
Timbre transfer technology has been making its way into released music for some years. In an early example, the track “Godmother” from Holly Herndon’s album PROTO, a percussive track by the producer Jlin is fed through a timbre transfer model trained on the human voice. The result is an uncanny beatboxed rendition, full of strange details and grainy artifacts.
“Godmother” has an exploratory quality, as if it is feeling out a new sonic landscape. This is a common quality to music made using timbral transfer. On A Model Within, the producer Scott Young presents five experimental compositions with just such a quality. Each explores a different preset model found in Neutone, capturing the unfamiliar interaction between human and machine.
Even before he’d encountered AI tools, a busy life made Young interested in generative composition approaches. When he started out making music, the producer recalls, “I spent a month making a tune. It was quite romantic. But my life in Hong Kong couldn't allow me to do that too much. And so I slowly attuned to Reaktor generators, to making sequences and stitching them together.”
Last year, the musician Eames suggested that he could speed things up further with generative AI. Young began exploring and came across RAVE, but struggled to get it to work, in spite of his background in software engineering. Then he discovered Neutone. “The preset models were so impressive that I eagerly began creating tunes with them. The results were mind-blowing. The output’s really lifelike.”
A typical fear surrounding AI tools is that they might remove creativity from music-making. Young’s experience with timbre transfer was the opposite. Timbre transfer models are - for now at least - temperamental. The sound quality is erratic, and they respond to inputs in unpredictable ways. For Young, this unpredictability offered a route out of tired music-making habits. “There's much more emphasis on serendipity in the making [process], because you can't always predict the output based on what you play.”
Once the material was generated, he still had to stitch it into an engaging composition - a process he likened to the editing together of live jazz recordings in an earlier era. “When using this generative approach, the key as a human creator is to know where to trim and connect the pieces into something meaningful that resonates with us.”
In the EP’s uncanniest track, “Crytrumpet,” Young feeds a recording of his crying baby daughter through a model trained on a trumpet. Moments like this neatly capture the sheer strangeness of AI technology. But timbral transfer is far from the only potential application of AI in plug-ins.
In March, Steinmetz co-organized the Neural Audio Plugin Competition alongside Andrew Fyfe of Qosmo and the Audio Programmer platform. The competition aimed to stimulate innovation by offering cash prizes for the most impressive entries. “As far as making neural networks inside plugins, it really hadn't been established yet,” says Steinmetz. “We need a way to encourage more people to work in this space, because I know there's stuff here to be done that's going to be really impactful.”
Of the 18 entries, some offered neural takes on conventional effects such as compression, and others proposed generative MIDI-based tools. Then there were the more surprising ideas. Vroom, a sound design tool, allows you to generate single sounds using text prompts. HARD is a novel “audio remixer,” enabling you to crossfade between the harmonic and rhythmic parts of two tracks independently. Everyone was required to open source their code, and Steinmetz hopes future plug-in designers will build on this work. He sees the start of a “movement of people interested in this topic.”
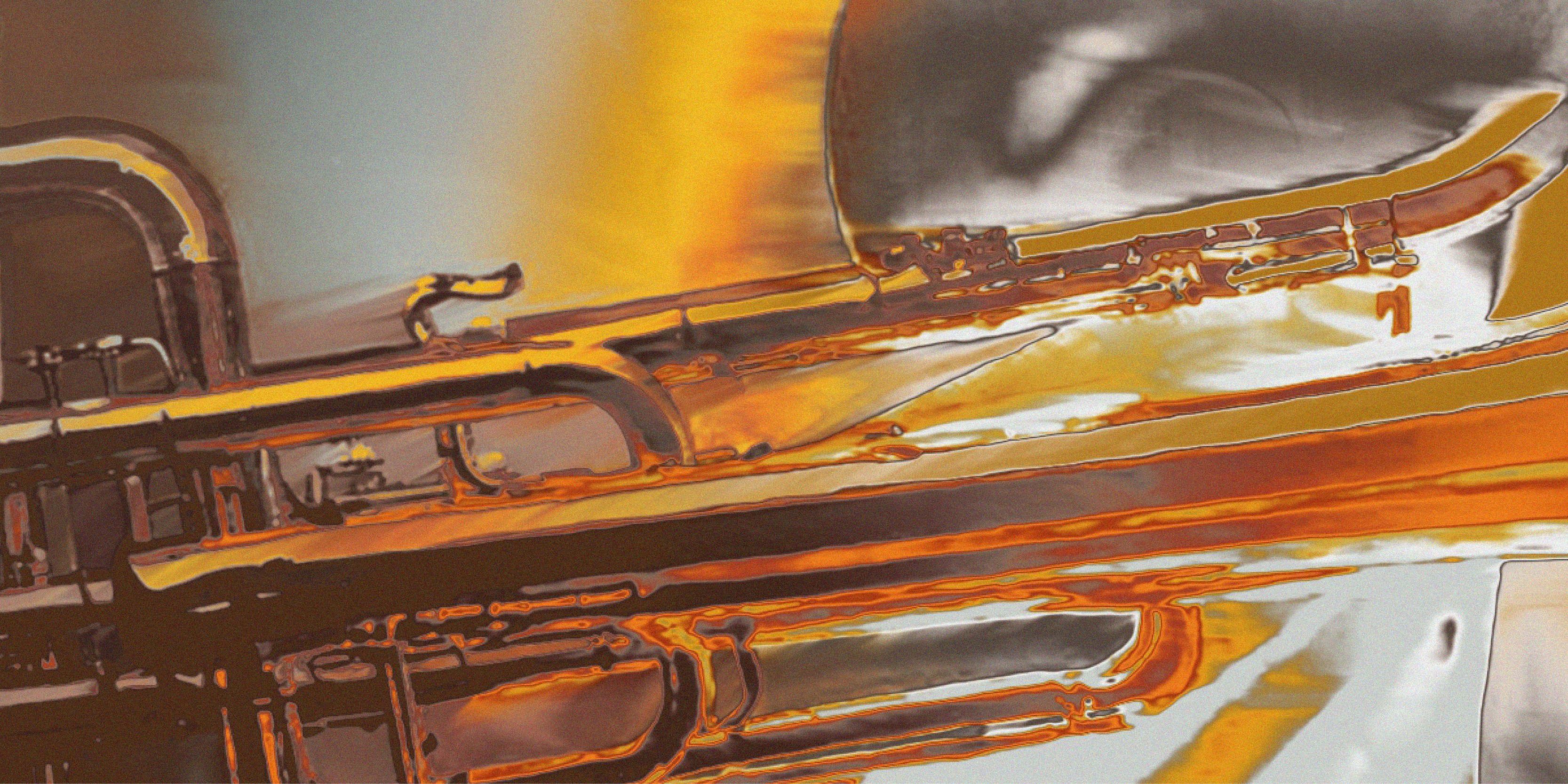
Analog modeling
So, AI can do new sounds. But it can also do old ones - perhaps better than we could before. Analog modeling is a cornerstone of the plug-in industry. According to some, AI could be its future. Plug-ins like Baby Audio’s TAIP (emulating “a 1971 European tape machine”) and Tone Empire’s Neural Q (“a well-known vintage German equalizer”) use neural network-based methods in place of traditional modeling techniques.
Baby Audio explain how this works on their website:
“Where a normal DSP emulation would entail ‘guesstimating’ the effect of various analog components and their mutual dependencies, we can use AI / neural networks to accurately decipher the sonic characteristics that make a tape machine sound and behave in the way it does. This happens by feeding an algorithm various training data of dry vs. processed audio and teaching it to identify the exact characteristics that make up the difference. Once these differences have been learned by the AI, we can apply them to new audio.”
Why use AI instead of traditional modeling methods? One reason is better results. Tone Empire claims that traditional circuit modeling “can never produce as authentic an analog emulation” as AI-based approaches.
Another is speed. Analog modeling using neural processing could potentially save a lot of time and money for plug-in companies. This means we might be looking at a proliferation of low-cost, high-quality analog models - no bad thing for producers who enjoy playing with new toys.
More radically, it means that modeling can be placed in the hands of music-makers themselves. This is already happening in the guitar world, via companies like TONEX and Neural DSP. Neural DSP’s Quad Cortex floor modeling unit comes with an AI-powered Neural Capture feature that allows guitarists to model their own amps and pedals. It’s simple: the Quad Cortex sends a test tone through the target unit and, based on the output audio, creates a high quality model in moments.
This presents exciting possibilities. Many of us have that one broken old pedal or piece of rack gear whose idiosyncratic sound we love. What if you could model it for further use in-the-box - and share the model with friends? Until now, modeling has mostly been the domain of technical specialists. It’s exciting to think what musicians might do with it.
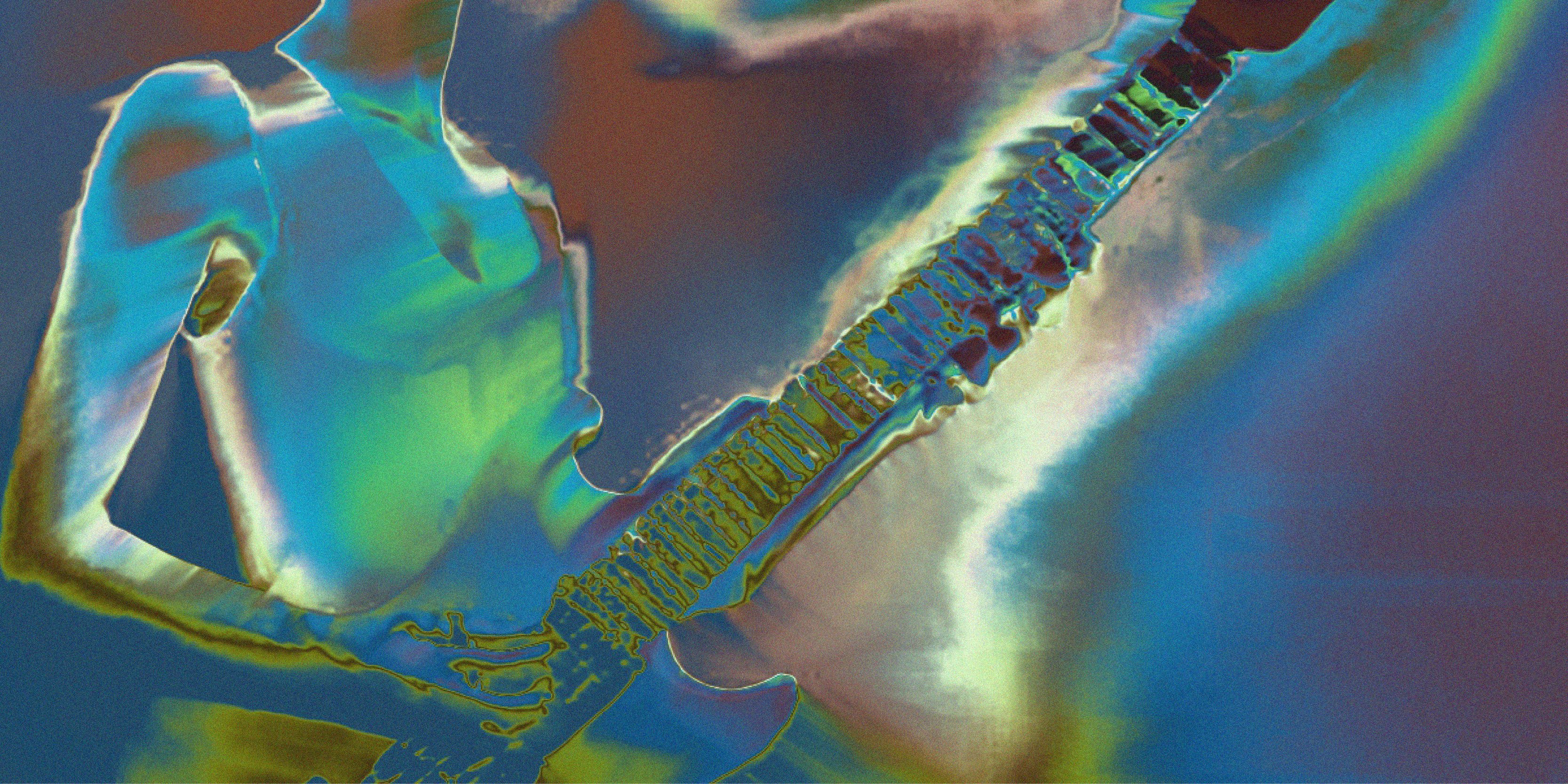
Democratizing music tech
This theme - of bringing previously specialized technical tasks into the hands of musicians - recurs when exploring AI music-making tools. For Steinmetz, analog modeling is just one application of deep learning technology, and not the most exciting. He invites us to imagine a tool like Midjourney or Stable Diffusion, but instead of producing images on command, it generates new audio effects.
“[This] enables anyone to create an effect, because you don't need to be a programmer to do it. I can search a generative space - just how I might search Stable Diffusion - for tones or effects. I could discover some new effect and then share that with my friends, or use it for my own production. It opens up a lot more possibilities for creativity."
We looked earlier at how certain production tasks may be automated by AI, freeing up musicians to focus their creativity in other areas. One such area might be the production tools they’re using. AI technology could enable everyone to have their own custom music-making toolbox. Perhaps making this toolbox as creative and unique as possible will be important in the way that EQing or compression is today.
Steinmetz envisions “the growth of a breed of programmer/musician/audio engineer, people that are both into the tech and the music side.” These people will either find creative ways to “break” the AI models available, or “build their own new models to get some sort of new sound specifically for their music practice.” He sees this as the latest iteration of a longstanding relationship between artists and their tools. “Whenever a [new] synthesizer is on the scene, there's always some musicians coming up with ideas to tinker with it and make it their own.”
Dryhurst also sees a future in artists building their own custom models, just as he and Herndon have done for PROTO and other projects. “I feel that is closer to how many producers will want to use models going forward, building their own ‘rig’ so to speak, that produces idiosyncratic results. I think that over time, we might also begin to see models themselves as a new medium of expression to be shared and experienced. I think that is where it gets very exciting and novel; it may transpire that interacting with an artist model is as common as interacting with an album or another traditional format. We have barely scratched the surface on the possibilities there yet.”
Read Part 2 of this article.
Text: Angus Finlayson
Images: Veronika Marxer
Have you tried making music with AI tools? Share your results and experience with the Loop Community on Discord. If you’re not already a member, sign up to get started.
AI and Music-Making Part 2: Tomorrow is the Question

Listen to this article.
This is Part 2 of our deep-dive into AI music-making. In Part 1, we learned what AI is; examined the challenges of applying AI technology to music-making; and explored uses of AI such as MIDI generation, timbral transfer, and analog modeling.
In this second part, we’ll take a broader look at AI’s impact on music-making. We’ll explore vocal deepfakes and speculate on the possibility of a ChatGPT for music. And we’ll examine some of the profound questions raised by AI, concerning creativity, originality, and what it means to be a musician.
Entering the deepfake era
The human voice has a unique position in our culture. No sound comes closer to expressing an authentic, unique self. Even when processed with effects like autotune, a voice is closely tied to a person - usually one person in particular. The singing or speaking voice is the ultimate sonic stamp of personhood. At least, it was.
What if we could have a voice without a human? Tools like Yamaha’s Vocaloid have long offered to synthesize voices from scratch. But the robotic results only really worked in situations where the artificiality was the point (such as virtual popstars like Hatsune Miku). AI tools are better at this task. With deep learning, it’s possible to generate voices that are so lifelike that they can trick the listener into “hearing” a person.
Take a plug-in like Dreamtonics’ Synthesizer V. You input the MIDI notes and lyrics, and select a voice bank with the desired characteristics (like Natalie, “a feminine English database with a voice that presents a soft and clear upper range as well as rich and expressive vocals on lower notes.”) And out comes a voice. The results are variable, but at their best could easily fool a casual listener. Indeed, they regularly do. Last year, Chinese company Tencent Music Entertainment revealed that it had released over 1000 songs featuring AI-generated voices.
The implications for the more commercial end of the music industry are profound. (Major labels must be intrigued by the idea of pop music without temperamental popstars.) But while voice synthesis using generic voice banks has many functional uses, it probably won’t replace human popstars any time soon. When we listen to our favorite singer or rapper, we’re enjoying that voice in particular: its timbre and grain, and its connection to a person who represents something we care about. Anonymous synthesized voices can’t compete with the aura of an artist.
But what if AI could imitate the voices we love? This April, the internet lost its mind over a new collaboration between Drake and The Weeknd, “Heart On My Sleeve.” So far, so normal - except the track was entirely fake, having been created using AI voice cloning technology by an artist calling themselves Ghostwriter. A few weeks previously, AI entrepreneur Roberto Nickson caused a similar stir when he used an AI tool to transform his voice into Kanye West.
AI voice cloning is a cousin of the timbral transfer technology we explored in part 1. But where timbral transfer plug-ins like Neutone sound like a tech in its infancy, voice cloning tools are getting shockingly good. This is true for speech as well as singing. Voice cloning AI company ElevenLabs caused consternation last year when they transformed Leonardo DiCaprio’s voice into Bill Gates, Joe Rogan, and others. They soon reported that pranksters were using their tool to make celebrities say offensive and inflammatory things.
We are entering a new era of “deepfakes.” Just as image generation tool Midjourney can convince us that the Pope wears Balenciaga, so too must we approach every recorded voice we hear with skepticism. But for electronic producers, this could present an opportunity. Sampling is a bedrock of electronic music, and sampled voices - whether full acapellas, sliced up phrases, or bursts of spoken word - are threaded through many dance music genres. This practice emerged from the more permissive sampling culture of the ‘80s and early ‘90s, but these days it can fall foul of litigious rights holders. What if AI allowed producers to “sample” their favorite vocalists without breaching copyright at all?
French club producer Jaymie Silk has long used sampled voices from movies or speeches in his music. On his 2021 Track “A President Is Just A Gangster With A Nuclear Weapon,” he got an iPad’s robotic text-to-speech function to recite the titular phrase. In late 2022, looking to push the idea further, he stumbled across an AI tool - he can’t remember which, but it could’ve been FakeYou - that offered text-to-speech with the voices of famous rappers and singers. He immediately saw its potential, and wanted to be the first to use the tool in a club context. (He was right to rush; a few months later, David Guetta “sampled” Eminem in the same way).
The result was Rub Music Vol. 1, an EP of pumping club tracks featuring vocal “samples” from artists like The Weeknd, Kendrick Lamar, and Tupac. The lyrics - written by Silk himself, of course - hint at the EP’s concerns: in “Illusions,” Tupac says, “What is real is not real… Everything is an illusion.” But while Silk’s EP expresses some deepfake angst, it also has the playful feel of a music-maker exploring an exciting new tool.
Voice cloning technology has improved since Silk made the EP. (He continued to use AI voices on his more recent release, Let’s Be Lovers). “It was basic at the time,” he remembers. “You couldn't modify or apply settings. You’d type something in, and you could be lucky.” The sound quality was also not ideal. In some cases - as in The Weeknd’s voice on “Artificial Realness” - extensive post-processing couldn’t remove the sibilant artifacts.
More recent voice cloning tech sounds better. It’s easy to imagine a tool combining the celebrity cast of FakeYou with the features of a voice synth like Synthesizer V. The result would be a mighty “sampling” toolbox, allowing you to have any popstar imaginable sing or rap words of your choosing.
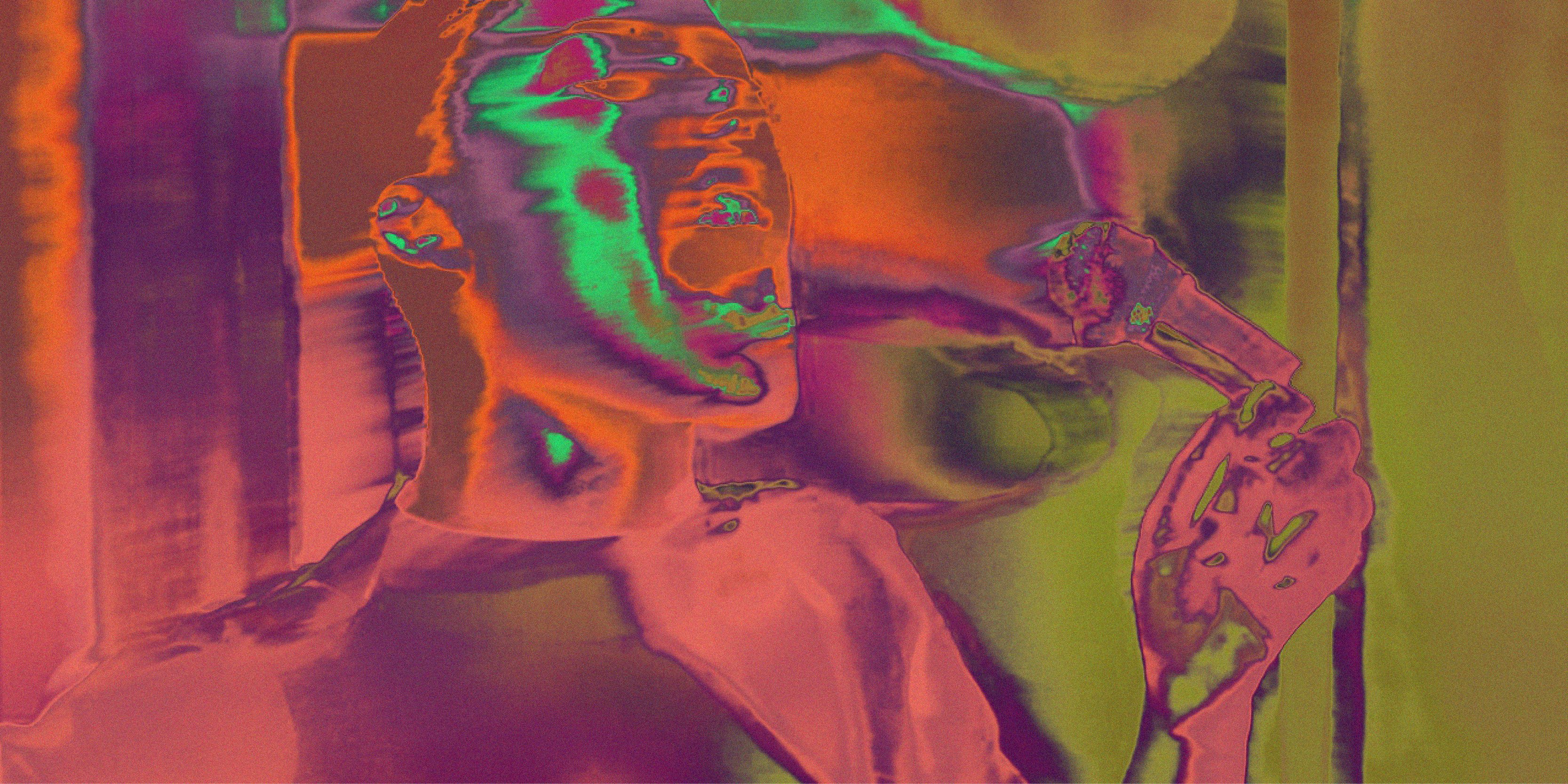
Who owns your voice?
But is this actually legal - or, for that matter, ethical? As we’ve discussed, a vocalist’s voice is the stamp of their personhood, and the main tool of their self-expression. Having this voice replicated a thousand times over could spell financial and creative ruin for many artists. Shouldn’t a vocalist get to decide who uses their sonic likeness?
Social context makes this question more pressing. Discussing the potential downsides of AI tools, Silk mentions the term “digital blackface,” which was leveled at Roberto Nickson for his Kanye video. Critics of Nickson, who is white, suggested that such tools provide a new way for white people to play with and profit off of black artists’ identities: a toxic dynamic at least as old as popular music.
If we consider voice cloning as a new form of sampling, then the dynamic that is emerging calls to mind injustices at the root of sampling culture. World-famous samples that have powered dance music for decades - such as the Amen and Think breakbeats - were performed by musicians who were never properly remunerated for the impact of their work. It’s easy to imagine AI voice tech having a similar exploitative dimension.
Some people saw this coming a long way off. Following her experiments with timbre transfer on 2019’s PROTO (discussed in Part 1), the musician Holly Herndon launched Holly+ in 2021. At the heart of the project is a high quality AI voice model of Herndon’s own voice: her “digital twin.” Users can interact with this model via a website, by uploading audio and receiving a download of the music “sung back” in Herndon’s “distinctive processed voice.” It’s like Jlin’s beats being sung on PROTO - but accessible to all, and in much higher quality.
As her statement on Holly+ explains, Herndon launched the project to address questions around “voice ownership,” and to anticipate what she sees as a trend of the future: artists taking control of their own “digital likeness” by offering high quality models of their voice for public use. This way, the artist can retain control over their voice, and perhaps profit from it. (Using Holly+ is free, but profits from any commercial use of the model go to a DAO, which democratically decides what to do with the money.)
According to Herndon, the voice cloning offered by tools like FakeYou may in fact violate copyright law - at least in the US. Providing context around “voice model rights,” Herndon cites legal cases back to the ‘80s in which public figures were protected “against artists or brands commercially appropriating their vocal likeness.” These precedents “suggest that public figures will retain exclusive rights to the exploitation of their vocal likeness for commercial purposes.” And indeed, UMG had the Drake x The Weeknd song taken down within a few days, arguing that music made with AI tools trained on their artists’ music violates copyright law.
A legal and ethical infrastructure needs to be built around these fast-developing tools. But, as with file-sharing in the 2000s, lawmaking may not return the genie to its bottle. Vocalists could find themselves competing - for attention, and perhaps for work - with their own digital likeness. In fact, it’s not only singers who feel this fear of replacement. Cheap or free-to-use image generation tools have become a tempting option for companies reluctant to pay a human illustrator. ChatGPT, meanwhile, fills professional copywriters with dread. The question is spreading through the creative industries and other white collar professions: Will AI take my job?
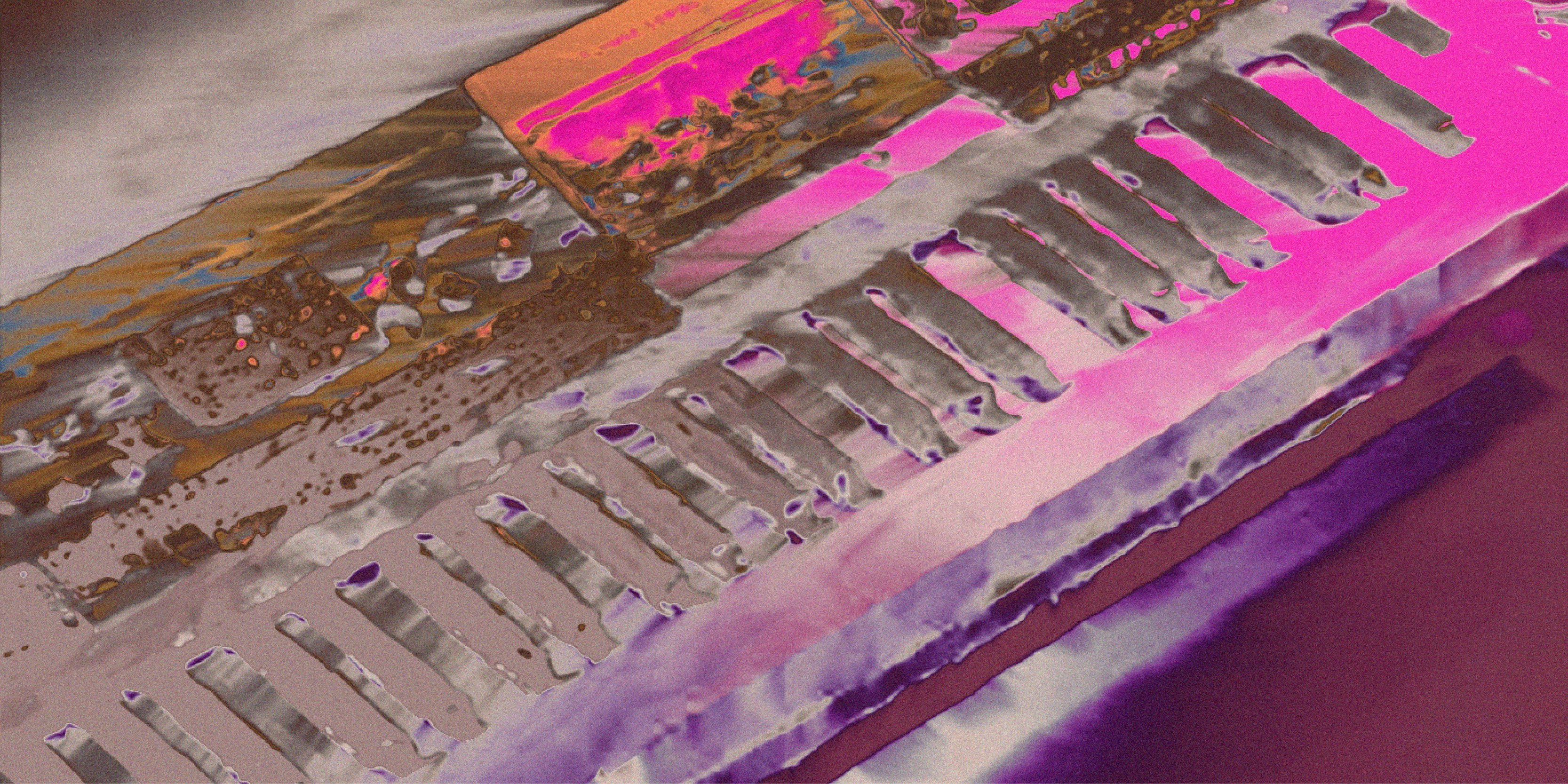
Automated composers
This brings us back to a question we touched on in Part 1. Tools such as ChatGPT and Stable Diffusion compete with human creators because of their sophistication and wide availability. An equivalent tool - powerful, good quality, and widely accessible - doesn’t yet exist for music. (We explored the reasons why in Part 1). But will it soon?
The answer from the specialists is a firm yes. Mat Dryhurst from Spawning mentions several organizations that are working on such a model. One is Google, whose MusicLM was introduced to the world at the beginning of this year, but isn’t yet publicly available. (Google started opening MusicLM to small groups of testers in May.) Another is HarmonAI, a music-focussed organization affiliated with Stability AI, the creators of the Stable Diffusion text-to-image model. HarmonAI involves The Dadabots, who have said that we can expect a tool from the organization “this year.”
To understand how such a tool might change the music-making landscape, we can start by looking at the less sophisticated AI music generators that already exist. While a “general” music model remains elusive for now, AI is already creating music in more limited contexts. In contrast to the tools explored in Part 1, these AI technologies aren’t typically designed to support existing music-making processes. Instead, they offer to remove the need for a skilled music-maker entirely - at least in certain situations.
Commercial composition is one such situation. Our world is lubricated by multimedia content, and there is an inexhaustible demand for soundtracks to adverts, podcasts, and social media posts. The makers of this content have a few options. They can commission a new composition at significant cost, or license a track from their favorite artist, probably for a hefty sync fee. Or they can source a cheaper soundtrack via a music library - the music equivalent of Shutterstock. But what if none of the music they can afford quite fits their needs? Or if their budget is tiny?
Here, AI products such as AIVA step in. AIVA began life in 2016 as an AI model trained to compose classical and symphonic music. (It was the first “virtual” composer to be recognized as such by a music rights society). The technology was made commercially available in 2019, and now presents itself as a “creative assistant,” promising to help you come up with “compelling themes for your projects faster than ever before.”
The process of generating a track is simple. The basic version is free to use. You hit “create a track” and start narrowing down your options. 12 preset styles, ranging from “20th Century Cinematic” to “Hip Hop,” set the frame in which the AI should work. You then pick parameters from a dropdown menu - key, tempo, instrumentation, and duration.
I chose a fast-paced “Fantasy” track performed by solo strings, and got 3 minutes of arpeggios with some disjointed melodic turns. It wouldn’t convince a close listener, but could work fine mixed at background level in a low-budget project. If I wanted, I could tweak the generation further in the MIDI-based editor mode. (The MIDI file can also be downloaded for further use).
AIVA can be effective with less sophisticated AI technology because it works in a tightly defined frame. Its preset styles and dropdown menus are a far cry from the anything-goes realm of natural text prompts. But when it’s formulaic, functional music you need, this method could work fairly well.
Should professional composers be worried? The answer probably echoes our discussion of mixing automation in Part 1. AI may soon be able to handle formulaic briefs where inspiration isn’t required (or desired). Higher-stakes projects will probably still benefit from a human’s creative vision. Perhaps a two tier system will emerge, with a human composer becoming a mark of high quality media. In other words, humans may become the premium choice.
This, at least, is one possible outcome suggested by generative AI composers. Other tools lead to a different conclusion. What if AI makes us all musicians?
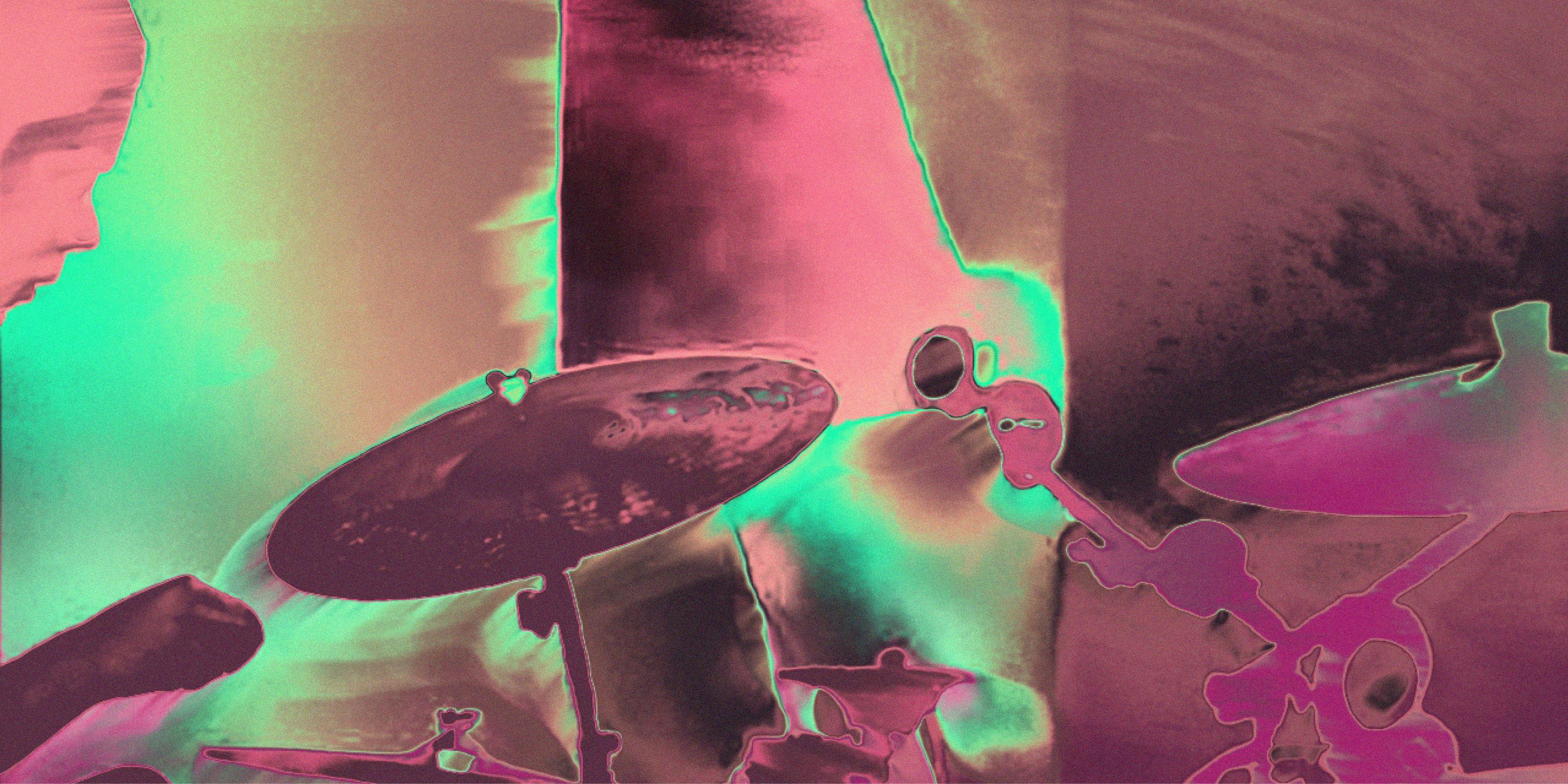
Everyone’s a music-maker
Boomy is an AI-based platform that invites you to “make original songs in seconds, even if you've never made music before.” It works similarly to AIVA. You navigate through dropdown menus of styles and sub-styles, and the AI generates a composition to your spec. You can then tweak the results with a simple editing suite.
Like AIVA, the tool gives you creative control within an extremely limited frame; and like AIVA, the results aren’t guaranteed to sound great. This hasn’t put off its user base. According to Boomy, the tool has been used to generate some 13 million songs, many of which have been uploaded to Spotify through the site and monetized by their creators.
Tools like AIVA and Boomy are no more than a glimpse of what might be coming. So far, their claim to supplant skilled music-makers is shaky even within the limited contexts that they address. But the rapid advances in AI in recent years should teach us not to dismiss this technology out of hand.
Google shared audio examples when introducing MusicLM, probably the most sophisticated text-to-music model so far presented to the public. Many of them are interesting mainly for their strangeness. (See, for example, the alien skronk prompted by the word “swing”). But others are more convincing. One 30 second clip - “a fusion of reggaeton and electronic dance music” - could be the start of a pretty compelling club track.
“The central challenge for music-makers will stay the same: how to break through the noise and reach an audience that cares.”
More recent examples shared online by GoogleLM’s testers demonstrate the same mix of the promising and the downright bizarre. But we should keep in mind the rapid progress of text-to-image tools over the past year, from smudgy sketches to hi-resolution deepfakes. Why shouldn’t it be similar for music? If this is where the technology is now, where might it be in a few years? Will it be possible for anyone to generate a decent-enough techno track in a few seconds?
“We live in the era of democratization of technology,” says Jaymie Silk. But this era started before AI came along. For decades, advances in technology have enabled more and more people to make music and share it with the world. It’s common to hear complaints that there is “too much” music being released. This doesn’t stop us from celebrating the artists who bring beauty and meaning into our lives.
Whether those artists can make a living is a different issue. The economics of music-making were tough long before AI came along, and AI could make them worse. The question of how musicians might make a living in an AI-powered age requires serious thought. But placing music-making into more people’s hands doesn’t mean there will no longer be special or profound music.
“When it becomes trivial for anyone to produce media to a certain level of sophistication, that just shifts our perception of what is banal, and background,” says Dryhurst. “It was once very laborious and technical to produce electronic music. Now anyone can buy a sample pack and some software, follow a tutorial on YouTube, and make something ok. That is not a bad thing, and that is often how people begin to learn how to express themselves. Automating that process even further just changes our baseline expectations, and says nothing about what artists are going to create to distinguish themselves from what you can now make with a click of a button. It will still take great technical skill, or inspiration, or luck, to create something that stands out. That has always been difficult, and will remain so.”
Jaymie Silk agrees. “There will be more shitty music, or more people doing music for fun.” But the central challenge for music-makers will stay the same: how to break through the noise and reach an audience that cares. “This part will not change. You still have to make good music, you still have to build a community.”
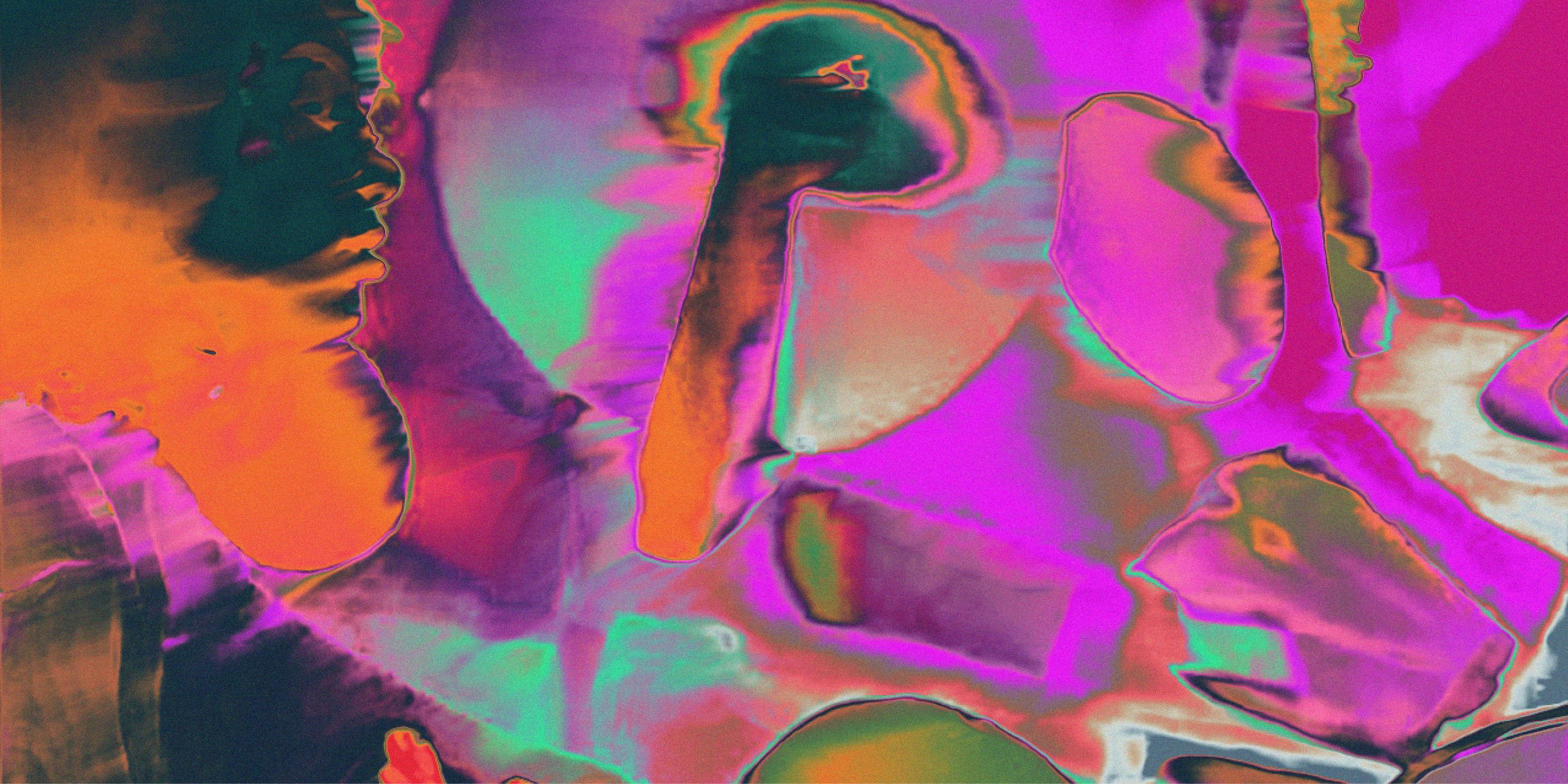
Spawning the future
Artists will use these new tools in expressive and imaginative ways, just as they have with new technologies in the past. In fact, they’re doing it already.
The London-based artist patten stumbled across Riffusion late last year. He was already familiar with generative AI from his work as a graphic designer. Riffusion caught his musician’s ear.
Launched towards the end of 2022, Riffusion was a hobby project that had an outsize impact. Rather than tackling text-to-music generation head-on, it piggybacks on the more successful text-to-image generation technology that already exists.
This works by “fine-tuning” - a process where you train an AI model on a specific kind of content to make it better at producing that content. The musicians Seth Forsgren and Hayk Martiros fine-tuned the text-to-image model Stable Diffusion on spectrograms (visual representations of the frequencies in a sound over time). These spectrograms can then be “read” and turned into audio. Voila: a text-to-image model you can hear.
Riffusion is a lot of fun to play with. You can feed it simple text prompts - “emotional disco,” “latent space vaporwave” - and it will play you an endless stream of iterating loops. You can also download your favorites. patten recognized the tool was more than just a toy. “After playing around with it for a short period of time, I came to realize that there was a lot that you could do with it. So I started pushing it and trying to see what I could get out of it.”
patten gathered material in a sleepless day-and-a-half binge of prompting and downloading. Later, he went back through what he’d collected, stitching the interesting parts into “fragmentary cloud-like pieces of music.” These pieces of music became Mirage FM, which patten claims is “the first album fully made from text-to-audio AI samples.”
It’s a beautiful, dreamlike record that doesn’t sound like anything else - though it flickers with hints of familiar styles. The content was entirely generated using Riffusion, but fans of patten will recognize his trademark aesthetic. A lot of the creativity, he says, came in the way he stitched the audio together. “Often it was these really tiny fragments that were spliced together into musical phrases and loops. I suppose this [album] was really about the edit as compositional expression.”
Dryhurst thinks that an approach like patten’s will soon be common among music-makers. “People will think nothing of generating passages of music to use in productions.”
One curiosity of Mirage FM is that, for all its boundary-breaking newness, there is a nostalgic quality to the music. This is helped by the tinny, artifact-riddled audio. (The Dadabots suggest that this may be down to “phase retrieval” issues caused by Riffusion’s spectrogram method.) patten likens this quality to cassette distortion or vinyl crackle. It’s an evocative comparison, particularly taken alongside the album’s tagline: “crate-digging in latent space.” We might think of AI tools as a portal into the future. But, trained as they are on a vast corpus of existing music, they’re also a window into our cultural past.
As with voice models, a comparison emerges between generative AI and sampling. Past generations of musicians dug through old music to find the one perfect sample; musicians of the future might search the “latent space” of an AI model for the choicest sounds. Only this time, the sounds might seem familiar, but they’re unique on each generation, and copyright-free.
The sampling comparison has been made before. The Dadabots made their name training AI models on artists they loved. A series of free Bandcamp releases captured the output of models trained on bands like Battles and Meshuggah. They have also presented their work as YouTube live streams – like RELENTLESS DOPPELGANGER, a “Neural network generating technical death metal, via livestream 24/7 to infinity.”
(They report “a range of responses” from the artists trained on their models. Some are “intrigued,” while other projects - like their fusion of Britney Spears and Frank Sinatra - have been flagged for copyright infringement.)
One such live stream, from 2021, came with a treatise on sampling. “Sampling serves an important use in music: there are sounds, feelings, messages, and reminders of history that can only be expressed through quotation.” But, the Dadabots wrote, copyright constraints limit musicians’ freedom to sample in their work. “Neural synthesis gives some of this ability back to musicians. We can now synthesize music that quotes a particular era, without sampling any previously published recording.”
The sampling comparison isn’t perfect, and some think it’s unhelpful. “Yes, of course, there's this technical possibility of circumventing the economic impact of wanting to sample,” says patten. “But I think there's huge potential for something more than that, which is less bound to the world of exchange and value, and is more about looking for forms of sonic experience that haven't existed before.” Dryhurst argues that we must “treat AI as a new paradigm” rather than falling back on old language and concepts. He and Herndon have coined a new term for the practice of generating AI audio for use in music: “spawning.”
But the idea of generative AI as consequenceless sampling helps us to address some of its ethical problems. As with voice models, the “copyright-free” tag doesn’t quite stick. Generative deep learning models are trained on data. The responses they give are based on patterns they’ve learned from this data. A text-to-image model like Stable Diffusion is trained on huge numbers of images, so that it can learn what makes a pleasing or accurate image - and produce one for us on demand. But where do these images come from?
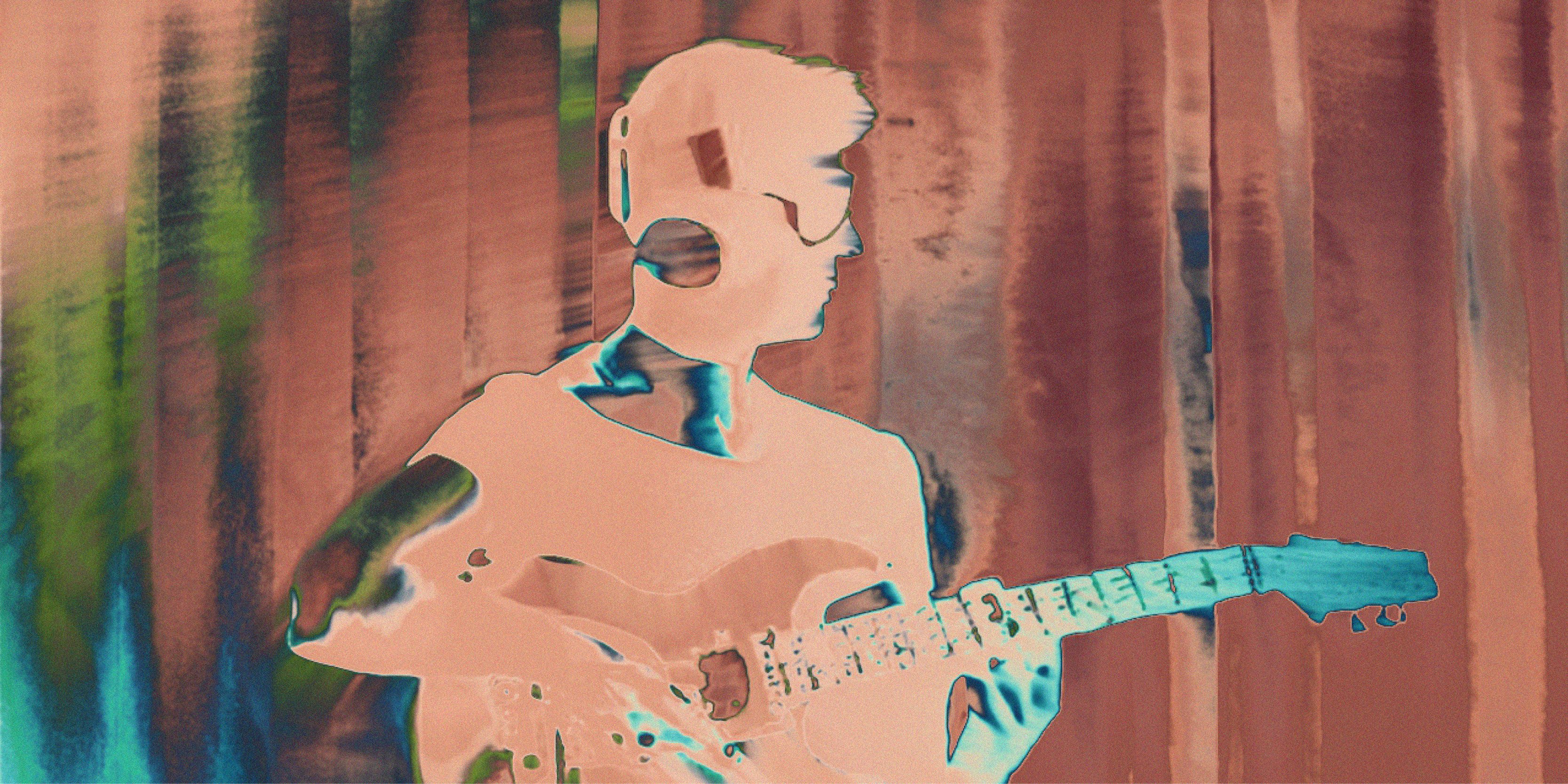
Copyright, ethics and originality
Stable Diffusion is trained on the LAION-5B image set, a massive trove of images scraped from the web. The images in LAION-5B are publicly available. But that doesn’t mean the creators of those images consented to their use in training AI models. Countless images from art sites such as Deviant Art have been used to train text-to-image models; that’s why the models are so good at generating illustration-like images in a style that we recognize.
Many of these artworks - and other images in datasets like LAION-5B - are copyrighted. According to current copyright law in the US and the EU, the inclusion of these artworks in a data set is allowed so long as it’s not being used for commercial purposes. But generative AI is a hugely profitable commercial enterprise - and the presence of these artworks in data sets is key to the technology’s appeal.
The ethical stakes start to look similar to those involved in sampling. Generating media from a deep learning model trained on non-consenting artists’ work isn’t so different from sampling their work without permission. In both cases, the original creators can’t give consent and don’t get paid.
This has led to a fightback from artists and rightsholders. A series of lawsuits are underway against AI models like Stable Diffusion, with the stock photo company Getty Images among the complainants. And there is heated debate around how to make data sets more consenting.
Dryhurst and Herndon have launched the tool Have I Been Trained? which allows artists to find out whether their work has been used in major data sets, and to opt out of its use in future. There is no legal mechanism to enforce this opt-out, but the idea is already having some success. Stability AI, the company behind Stable Diffusion, have said they will honor the opt-out (which now encompasses 80 million images) in the next iteration of their model.
This war over intellectual property is being waged over images. What about audio? The music industry’s complicated ownership structures make it more resistant to the creation of consent-less data sets. In fact, some say this is partly why generative AI music models lag behind image and text: it’s harder to get the data to train them.
“The music industry has a mind bogglingly complex structure, and the layers of organizations required to ensure enforcement of copyrights can result in cautiousness about new means of distributing music,” explain The Dadabots. “Even if an artist is excited about AI, it might not be solely up to them if the generated music can be sold. Popular artists often do not fully own their music and are sometimes not able to give permission for its use without consulting labels or publishers.”
It’s surely no bad thing if technology has to wait a little while legal and ethical frameworks catch up. The hope is that this is reflected in forthcoming generative models. HarmonAI, for example, are taking measures to seek consenting data for their forthcoming Dance Diffusion model. Meanwhile, Have I Been Trained? intends to expand its functionality to include audio. “The fundamentals we are putting in place will work across media type,” says Dryhurst.
Beyond the issue of consent, AI’s reliance on data sets raises questions about its scope. Critics will say that this is a fundamental limitation of AI. Trained on existing human creations, an AI model can’t do anything new - just regurgitate ideas we’ve already had, albeit in new combinations. In this depiction, musicians who use AI could become mere curators, reshuffling familiar ingredients in an increasingly derivative cultural soup.
On closer inspection, though, the line between “curation” and “creation” isn’t so clear. “In music there are only so many instruments that exist, there are only so many chord progressions, only so many ways to put them together,” says Christain Steinmetz. “So a band is actually kind of curating music theory, picking the parts that they like, and packaging them into some creative material.”
patten takes the idea further. “When we say, ‘[the AI] is not doing anything new, because it's derived from existing material,’ you’ve got to think: What is it that we're doing in this conversation now? We're not inventing a whole system of linguistic devices to express ourselves. We're using a language that we both share and understand, with its various histories.” In this way, for patten, AI tools open up profound questions about what creativity and originality really are. “There's this incredible opportunity to look at some age-old questions about the nature of consciousness, humanity, creativity. And to reflect on what it is that we're doing when we're doing these things - and what makes us human.”
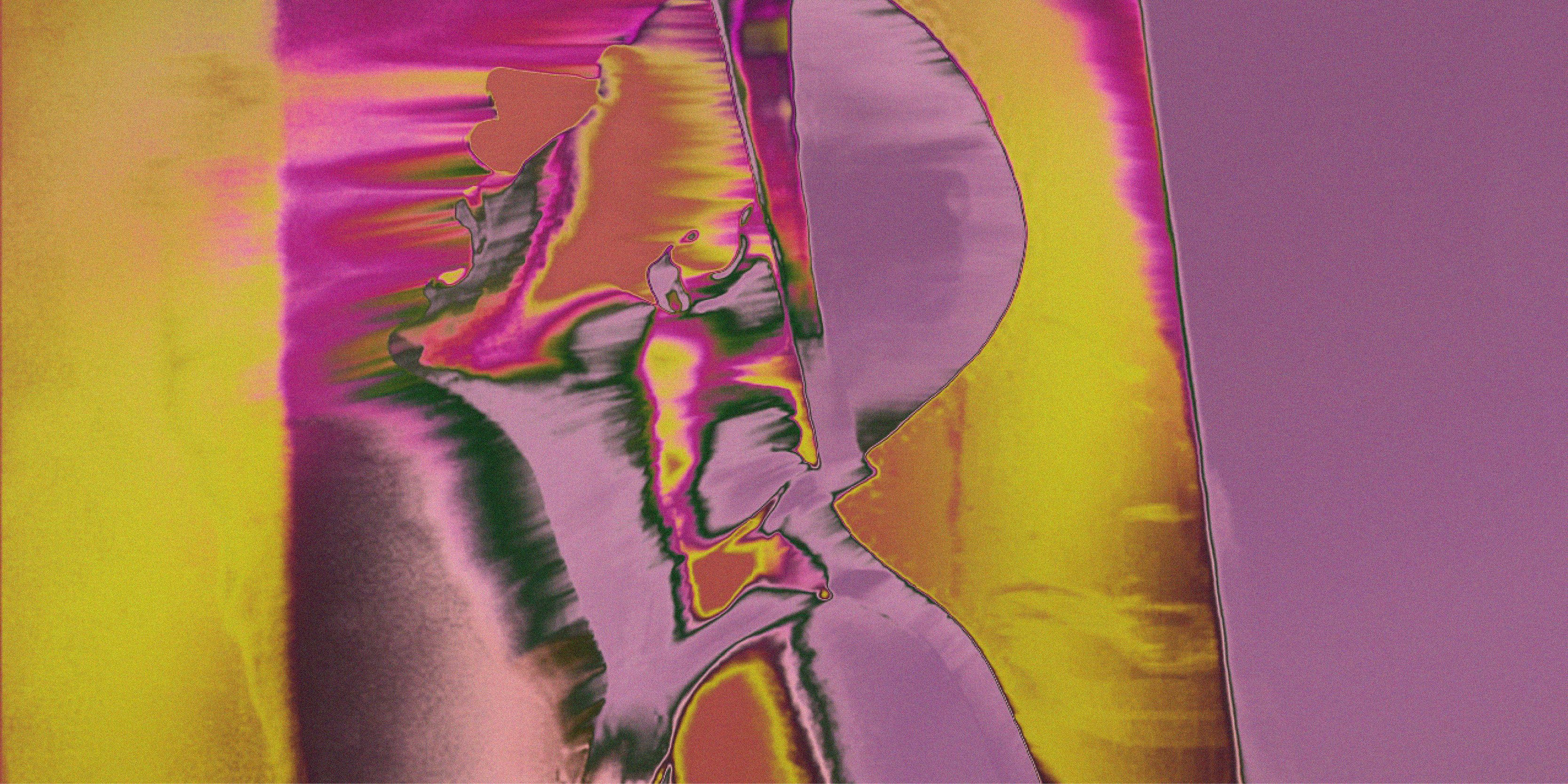
Conclusion: Money, Automation and Transitioning To The Future
In these articles we’ve looked at a number of ways that AI technology could change music-making. We’ve covered a broad spectrum of activities, from taking over technical mix tasks to generating MIDI, placing famous voices in producers’ hands, and “spawning” passages of audio and entire compositions. What unifies these different uses of AI? In each case, the AI is doing something that would have required human effort before. In other words: all of them are forms of automation.
The drive to automate has been fundamental to the last few centuries of human history. Automation means using machines to make products quicker than a human can, or getting computers to do complicated sums for us. By reducing the human effort required in a process, automation lowers costs and increases productivity. That means more money for whoever owns the automating machine.
This gives us a clue as to the driving force behind new AI technologies. It takes enormous resources (not to mention a hard-to-calculate environmental cost) to train vast deep learning models like ChatGPT and Midjourney. It is typically large tech companies that can afford to bankroll these models, and it is these companies that will reap the rewards (or so they hope).
AI isn’t simply a story about monopolistic tech giants. There are many creative people working on AI music tools, driven by a spirit of discovery and a thirst for new sounds. But in the scheme of things, AI music is a sideshow to the main event: the automation of vast swathes of our economy.
History teaches us that automation is a painful process. Hard-earned skills become redundant or devalued; livelihoods are lost; cultures and communities are upended. Cushioning the impact is a political challenge, raising questions about how we organize our societies and who and what we value. Battles over the meaning and implications of AI technology are already being fought, and they will intensify in the coming years.
But looking at history, we can see that these upheavals have never spelled doom for musical creation itself. Such moments shift the frame of what we consider to be music, and what we consider to be a musician. Some musical traditions lose their relevance, but previously unimaginable new ones emerge. Silicon Valley didn’t have techno music in mind when the microprocessor was invented. But a chain of events was set in motion that led to mass market audio synthesis, home computing, and a whole new way of making music.
The important thing to remember is that technology didn’t make the music happen. People did, by adapting and responding to the moment they lived in.
“One of the challenges we face being in the present is the inability to see it as a transition,” says patten. “When we describe what it is to be a musician, it's within a really specific temporal field, when things are a certain way.” Music-making technologies “come and go. Like the electric guitar, the CD, turntables. All of these things create and carve out circumstances and behaviors that are very real, but they're never static. We should consider that the way things are right now isn't necessarily the way that they're always going to be, or the best [way]. So, the death of what we consider now to be a musician: we don't have to view that as a negative.”
Read Part 1 of this article.
Text: Angus Finlayson
Images: Veronika Marxer
Have you tried making music with AI tools? Share your results and experience with the Loop Community on Discord.
Out now: Free Live 11.3.10 update
Ableton Live 11.3.10 is now available for Live and Push.
This free update improves the speed, accuracy and performance of auto-warping with different types of audio material. It also includes a variety of bugfixes and improvements to stability for the standalone version of Push.
Check out the release notes* for more information on everything in Live 11.3.10. If you already own a Live 11 license, the update is free for you.
Live users can download the free update here.
If you own the standalone version of Push, you can update from the Software tab in Push’s settings menu.
*Release notes are only available in English.
Coming Soon: A new public beta for Push
In the coming weeks, we’ll release the next beta for Push. It brings improvements to working with samples and better integration with external gear.
Want to be among the first to test these new features? Join the Ableton Beta Program for free.*
*Note: The Ableton Beta program is only available in English.
The standalone Push is back in stock
If you want to make music laptop-free and enjoy the latest Live updates on Push’s expressive pads, you can now order the standalone version in all available markets. Get fast, free shipping and pay in monthly installments with no extra fees. Shop now ›
What’s new in Ableton Note

Tap into a new creative habit with Note, Ableton’s iOS app for musical sketching.
Note is always evolving. We’ve listened to your feedback and added a bunch of new features to help you to quickly sketch out ideas, find your favorite sounds, and be ready to capture new ones whenever inspiration strikes. Check out what’s new and stay tuned – there's more coming soon.
Control with MIDI
Prefer playing with keys? You can now connect any USB Class Compliant MIDI controller via Bluetooth or USB for another way to play drums, sketch melodies and create chords in Note.
Search for new sounds
There are lots of versatile new synth sounds to play with, including a selection based on Drift, Live’s new analog-flavored synth. They’re easier to find, too – you can use the search bar in Note’s browser to find the presets or samples you’re looking for.
Save presets
Create your own presets and save them to your User Library, then use the new favorite and filter functions to pull them up faster when you want to use them again.
Reverse, copy and paste samples
Flip drums and melodic samples – perfect for fills, ambient textures and many other sound design tricks. The Drum Rack also lets you copy and paste pads to add further variation to your sound palette, or to slice longer samples across multiple pads.
Import samples
You no longer have to leave the app to import your samples – try importing directly from Note’s browser instead.
Convert videos to sound
You can also import the sound from your favorite videos, straight from your Photos app. So whether you just captured a great moment on camera or took a screen recording of a viral video, no sound is off limits.
Adjust note length
Transform staccato to sustained notes and back again using the Note Length buttons, plus nudge them around the grid with the improved Operations tab.
Improved accessibility
If you use iOS Voiceover, you’ll find improved functionality and better access when using Note with a screen reader – it’s available in more languages too. Try the High Contrast theme in the app settings to make Note easier to use in any conditions.

The new High Contrast theme
Home screen and lock screen widgets
Add widgets to keep your Sets within reach, or create new ones directly from the iOS home screen or lock screen and start sampling immediately.

We hope you’re enjoying Note’s new features. To take a closer look at everything that’s been added, check out the full release notes*. As always, feel free to send us your feedback via the app settings.
*Release notes are available in English only
Experience Live 12 and Push 3 In-Person
Around the announcement of Live 12, a series of creative meetups will be taking place worldwide to give like-minded music makers an opportunity to come together and discover new ways of making music.
Meetups are a place to exchange ideas and technical tips, explore different setups, and make new connections. At some events, you’ll get to see the new features of Live 12 or Push 3 in action – and others even give you the chance to try them for yourself.
Check the listings below to find your nearest event, see what’s on offer, and get involved. We’ll be adding more in the coming weeks – so please check back if you’re waiting for one in your area.
Upcoming
Australia
Cairns
Date: 3rd April
Location: NorthSite Studios
Details and RSVP: Facebook
Event topic: Live 12 and Push 3
Mexico
Querétaro
Date: 17th April
Location: Tec de Monterrey University
Details and RSVP: TBC
Event topic: Live 12
Thailand
Bangkok
Date: 30th March
Location: In Ear Beat
Details and RSVP: Facebook
Event topic: Live 12
Past events
United States
Brooklyn, NY
Date: 21st - 22nd October
Location: Brooklyn Synth Expo
Details and RSVP: Eventbrite
Chicago
Date: 30th November
Location: Spybar
Details and RSVP: Eventbrite
Event topic: Live 12 and Push 3
Minneapolis
Date: 1st December
Location: Slam Academy
Details and RSVP: Eventbrite
Event topic: Live 12 and Push 3
New York
Date: 2nd December
Location: 343 Labs
Details and RSVP: Eventbrite
Event topic: Live 12 and Push 3
Austin
Date: 7th December
Location: Dub Academy
Details and RSVP: Eventbrite
Event topic: Live 12 and Push 3
Los Angeles
Date: 12th December
Location: Musician’s Institute Hollywood
Details and RSVP: Eventbrite
Event topic: Live 12 and Push 3
Pasadena, CA
Date: 10th February
Location: Ableton Inc. Office
Details and RSVP: Eventbrite
Event topic: Live 12 and Push 3
Knoxville, TN
Date: 21st - 24th March
Location: Big Ears Festival
Details and RSVP: Website
Event topic: Live 12 and Push 3
Pittsburgh, PA
Date: 24th March
Location: Mary Pappert School of Music, Room 206
Details and RSVP: Facebook
Event topic: Live 12
United Kingdom
Manchester
Date: 14th October
Location: Hallé St Peter's
Details and RSVP: Website
London
Date: 16th October
Location: SAE London
Details and RSVP: Website
London
Date: 28th October
Location: Tileyard London
Details and RSVP: Eventbrite
London
Date: 16th November
Location: Tileyard London
Details and RSVP: Eventbrite
Glasgow
Date: 24th November
Location: Subsine Academy
Details and RSVP: Website
Event topic: Live 12 and Push 3
Bristol
Date: 25th November
Location: Dareshack
Details and RSVP: Eventbrite
Event topic: Push 3 only
Manchester
Date: 2nd December
Location: Islington Mill
Details and RSVP: Eventbrite
Event topic: Push 3 only
Glasgow
Date: 7th December
Location: Subsine Academy
Details and RSVP: Eventbrite
Event topic: Push 3 only
Edinburgh
Date: 25th March
Location: Leith Depot
Details and RSVP: Eventbrite
Event topic: Live 12
Australia
Melbourne
Date: 21st October
Location: MESS
Details and RSVP: Website
Sydney
Date: 27th November
Location: TAFE NSW, Ultimo Campus, Building M
Details and RSVP: Facebook
Event topic: Live 12 only
Melbourne
Date: 5th December
Location: Arts Centre Melbourne
Details and RSVP: Facebook
Event topic: Live 12 only
Cairns
Date: 14th December
Location: CQUniversity Cairns - Level 1 Studios, Cnr Shields and Abbott St
Details and RSVP: Facebook
Event topic: Live 12 and Push 3
Adelaide
Date: 24th March
Location: Immersive Light & Art Adelaide
Details and RSVP: Website
Event topic: Live 12 and Push 3
Brisbane
Date: 24th March
Location: Diffuszed Studios
Details and RSVP: Facebook
Event topic: Live 12
Canada
Toronto
Date: 5th December
Location: Hart House Building
Details and RSVP: Eventbrite
Event topic: Live 12 and Push 3
Montreal
Date: 12th December
Location: Bar Le Ritz
Details and RSVP: Eventbrite
Event topic: Live 12 and Push 3
Windsor, ON
Date: 25th March
Location: University of Windsor, Alan Wideman Building
Details and RSVP: Facebook
Event topic: Live 12
New Zealand
Auckland
Date: 28th October, 11am - 1pm
Location: Rockshop Auckland Central Digital
Details and RSVP: Instagram
Austria
Vienna
Date: 17th October
Location: Praterstrasse
Details and RSVP: Eventbrite
France
St Etienne
Date: 2nd - 3rd November
Location: Positive Education Festival
Details and RSVP: Eventbrite
Montpellier
Date: 9th November
Location: Blackout
Details and RSVP: Facebook
Montpellier
Date: 10th - 11th November
Location: Fastlane
Details and RSVP: Eventbrite
Rouen
Date: 16th November
Location: Le 106
Details and RSVP: Eventbrite
Bordeaux
Date: 22nd November
Location: Art School
Details and RSVP: Eventbrite
Event topic: Push 3 only
Strasbourg
Date: 30th November
Location: Shadock
Details and RSVP: Eventbrite
Event topic: Push 3 only
Paris
Date: 5th December
Location: FGO Barbara
Details and RSVP: Eventbrite
Event topic: Push 3 only
Marseille
Date: 8th December
Location: Cabaret Aléatoire
Details and RSVP: Eventbrite
Event topic: Push 3 only
Annecy
Date: 14th December
Location: Brise Glace
Details and RSVP: Eventbrite
Event topic: Push 3 only
Grenoble
Date: 15th December
Location: La Belle Électrique
Details and RSVP: Eventbrite
Event topic: Push 3 only
Lyon
Date: 16th December
Location: La commune
Details and RSVP: Eventbrite
Event topic: Push 3 only
Germany
Berlin
Date: 11th October
Location: Ableton office
Details and RSVP: Eventbrite
Hamburg
Date: 13th - 14th December
Location: SAE Institute
Details and RSVP: Eventbrite
Event topic: Push 3 only
Berlin
Date: 6th - 29th February
Location: Ableton Office
Details and RSVP: Eventbrite
Event topic: Push 3
Italy
Turin
Date: 9th November
Location: Martin Pas Synth Shop
Details and RSVP: Eventbrite
Mannheim
Date: 9th November
Location: Musikpark Mannheim
Details and RSVP: Eventbrite
Turin
Date: 11th -12th November
Location: Soundmit Turin
Details and RSVP: Website
Bologna
Date: 14th November
Location: Creative Hub
Details and RSVP: Eventbrite
Verona
Date: 17th November
Location: ALOUD
Details and RSVP: Eventbrite
Event topic: Push 3 only
Milan
Date: 21st November
Location: SAE Milan
Details and RSVP: Eventbrite
Event topic: Push 3 only
Rome
Date: 29th November
Location: Fonderie Sonore
Details and RSVP: Eventbrite
Event topic: Push 3 only
Rome
Date: 30th November
Location: Hinkypunk Studio
Details and RSVP: Eventbrite
Event topic: Push 3 only
Naples
Date: 15th December
Location: NUT Academy
Details and RSVP: Eventbrite
Event topic: Push 3 only
India
Pune
Date: 11th - 13th March
Location: ADYP University
Details and RSVP: Website
Event topic: Live 12 and Push 3
Bangalore
Date: 11th - 13th March
Location: CMR University
Details and RSVP: Website
Event topic: Live 12 and Push 3
Delhi / Gurugram
Date: 12th - 13th March
Location: The NorthCap University
Details and RSVP: Website
Event topic: Live 12 and Push 3
Japan
Tokyo
Date: 20th - 21st October
Location: Ableton Tech Lounge
Details and RSVP: Website
Osaka
Date: 18th November
Location: Kansai Modular Festival
Details and RSVP: Website
Event topic: Push 3 only
Osaka
Date: 19th - 25th November
Location: Shimamura Umeda
Details and RSVP: Website
Event topic: Push 3 only
Tokyo
Date: 25th - 26th November
Location: Tokyo Modular Festival
Details and RSVP: Website
Event topic: Push 3 only
Nagoya
Date: 26th November
Location: Beat Grand Prix
Details and RSVP: Website
Event topic: Live 12 and Push 3
Netherlands
Amsterdam
Date: 17th - 21st October, 11am - 6pm
Location: ADE Lab (Het Groene Paleis - Rokin 65)
Details and RSVP: Website
Amsterdam
Date: 20th October, 11am - 4pm
Location: Armada University (Lynk & Co - Rokin 75)
Details and RSVP: Website
Amsterdam
Date: 18th November
Location: SAE Amsterdam
Details and RSVP: Website
Event topic: Push 3 only
Helmond
Date: 30th November
Location: Night of the Nerds
Details and RSVP: Website
Event topic: Push 3 only
Utrecht
Date: 6th December
Location: No Mans Land
Details and RSVP: Website
Event topic: Push 3 only
Nijmegen
Date: 12th December
Location: Night of the Nerds
Details and RSVP: Website
Event topic: Push 3 only
Singapore
Singapore
Date: 6th December
Location: City Music 3.0
Details and RSVP: Website
Event topic: Live 12 and Push 3
Spain
Barcelona
Date: 16th November
Location: SAE Barcelona
Details and RSVP: Eventbrite
Valencia
Date: 25th November
Location: Reb/el Cabanyal
Details and RSVP: Eventbrite
Event topic: Push 3 only
Madrid
Date: 28th November
Location: SAE Madrid
Details and RSVP: Eventbrite
Event topic: Push 3 only
Bilbao
Date: 30th November
Location: Sala Konekta - La Perrera
Details and RSVP: Eventbrite
Event topic: Push 3 only
Madrid
Date: 14th December
Location: DJP Music School
Details and RSVP: Eventbrite
Event topic: Push 3 only
Santiago de Compostela
Date: 16th December
Location: Garaxeland
Details and RSVP: Eventbrite
Event topic: Push 3 only
South Korea
Gunsan
Date: 2nd December
Location: Howon University
Details and RSVP: Website
Event topic: Live 12 only
Seoul
Date: 23rd March
Location: Seoul Community Radio
Details and RSVP: Website
Event topic: Live 12
Thailand
Bangkok
Date: 8th December
Location: ProPlugin Flagship
Details and RSVP: Facebook
Event topic: Live 12 and Push 3
What’s coming in Live 12
Live 12 brings new ways to explore who you are as a music maker.
Kick-start melodies and rhythms with MIDI Generators, and take them off the beaten track with Transformations. Whip up wild sound experiments and shape the results as you go with Meld, Roar and Granulator III. See everything you need with Stacked Detail Views, find the sample you’re looking for with Sound Similarity Search, and much more.
If you want to try the Live 12 public beta, you can now join the waiting list – it may take some time to receive access, so we kindly ask for your patience.
The 10 best drum breaks of all time (and how to recreate them)
What are drum breaks?
Drum breaks originated as drum solos within funk and soul tracks – a moment for drummers to shine with their rhythmic prowess and show off new and unique drum patterns. Later, these solos caught the ear of hip-hop producers who began sampling these breakbeat loops while programming drums, laying a foundation for MCs to rap over. This practice not only created new music, but also helped craft the sound and rhythmic vibe of the entire drum and bass genre, weaving its way into various other styles of electronic music along the way.
Drum breaks transitioned from mere solos to become a fundamental element of modern music, demonstrating their versatility and enduring appeal across the musical spectrum.
10 best drum breaks of all time
Now that you know what drum breaks are and where they came from, let’s take a look at how to recreate ten of the most famous drum breaks. For these demonstrations, we will be using several of the drum kits found in the Rudiments Kontakt instrument.
Rudiments is perfect for crafting breakbeats because it’s full of modern sounding hip hop drum kits and patterns, but it still has plenty of that retro grit we’ve all come to love.
Also, I’ve included links to MIDI files for each drum break so you can easily download them for free and use them with the Rudiments drum kits in your own productions. I hope they help to spark new inspiration in your beat making sessions!